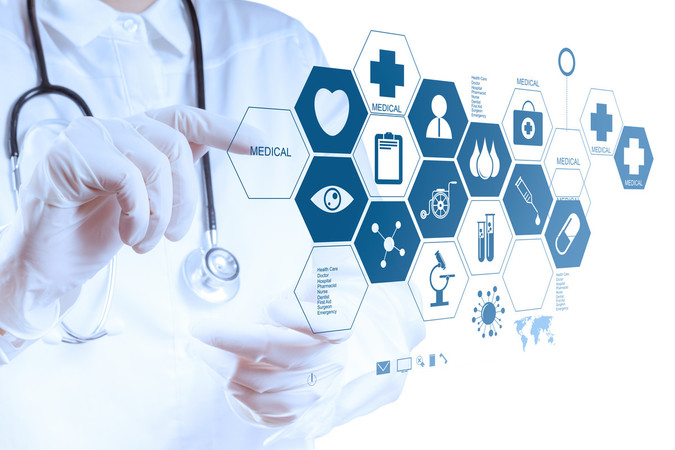
Intelligent lung support system for acute respiratory failure |
Medical Need
Mechanical ventilation (MV) represents one of the most relevant therapies to support organ function in the intensive care unit, as well as in the operating room. Inappropriate mechanical ventilation settings in intensive care patients are associated with organ damage. Currently, there are different strategies to protect the lungs from injury by the ventilator. Yet, settings may differ substantially regarding to the mechanical energy transferred to lungs, and its distribution across the parenchyma. Artificial intelligence can be used to learn from experts in mechanical ventilation in an attempt to accomplish lung support with reduced risk of damage.
This project aims to develop and implement a wireless and artificial intelligence-based system for mechanical ventilation of endotracheally intubated patients that is capable of guiding MV within safer therapy corridors for longer periods in an attempt to reduce ventilator induced lung injury.
mechanical ventilation, respiratory failure, ventilator induced lung injury, extracorporeal membrane oxygenation, artificial intelligence, wireless communication
Project team members |
High-tech |
Institute for Applied Informatics (InfAI) Dresden
Abstract |
Intensive care patients with acute respiratory failure usually need support of lung function, which is accomplished with mechanical ventilation and, in most severe cases, extracorporeal gas-exchange. Although mechanical ventilation is a life-saving therapy, it has the potential to worsen lung injury and impair the haemodynamics. Currently, there are different strategies to protect the lungs from injury by the ventilator. Yet, settings may differ substantially regarding to the mechanical energy transferred to lungs, and its distribution across the parenchyma. Variables at the ventilator and extracorporeal lung support device can be set automatically using optimization functions and clinical recommendations, but the handling of experts may still deviate from those settings depending upon clinical characteristics of individual patients. Artificial intelligence can be used to learn from those deviations as well as the patient’s condition in an attempt to improve the combination of settings and accomplish lung support with reduced risk of damage. The project proposed herein aims at developing a hybrid mechanical ventilator/extracorporeal lung support device, where elements communicate wireless, using artificial intelligence-based algorithms to improve the care of invasively mechanically ventilated patients with acute respiratory failure.