Deciphering Metastasis with Multimodal Artificial Intelligence Foundation Models
DECIPHER-M
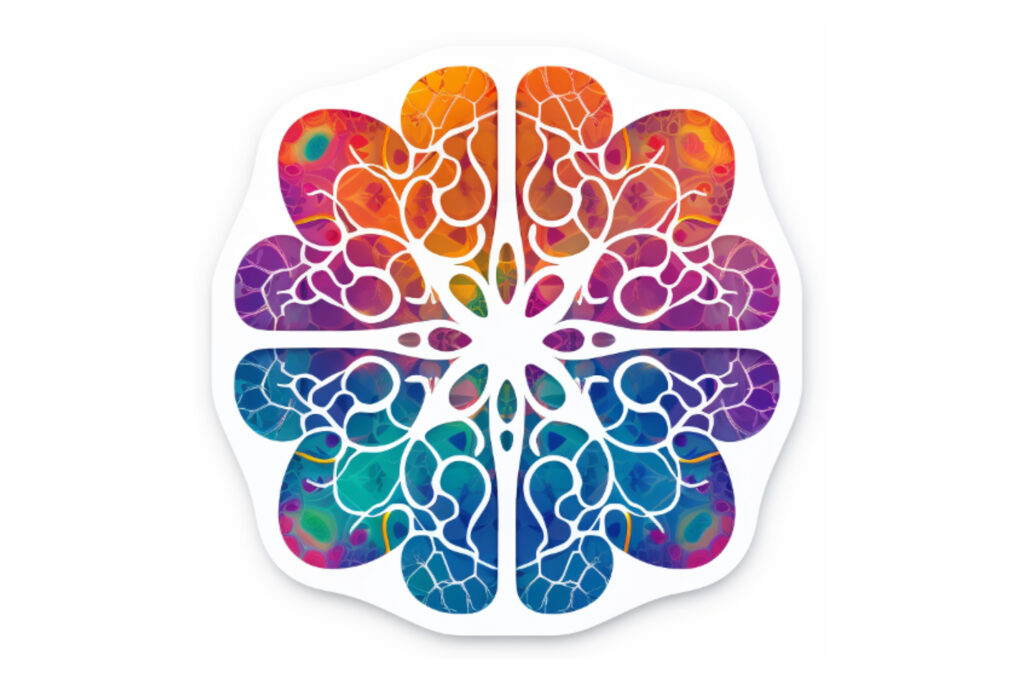
About DECIPHER-M
Cancer metastasis is a complex process whose understanding requires a multidisciplinary approach. Our consortium aims at integrating a wide variety of clinical data types with multimodal AI models, aimed at deciphering the biological mechanisms of metastasis, improving early detection and ultimately facilitating effective therapeutic interventions.
Subprojects
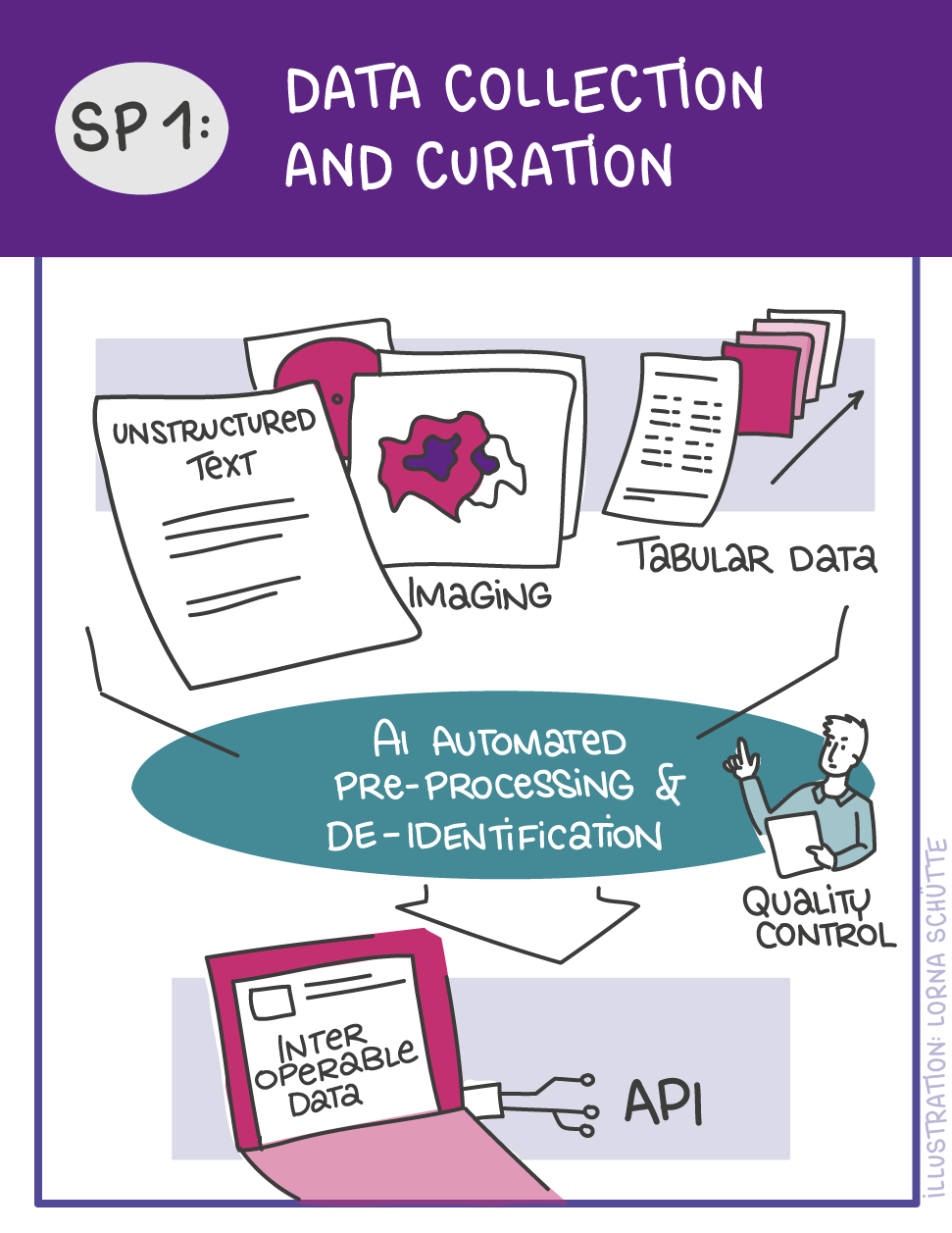
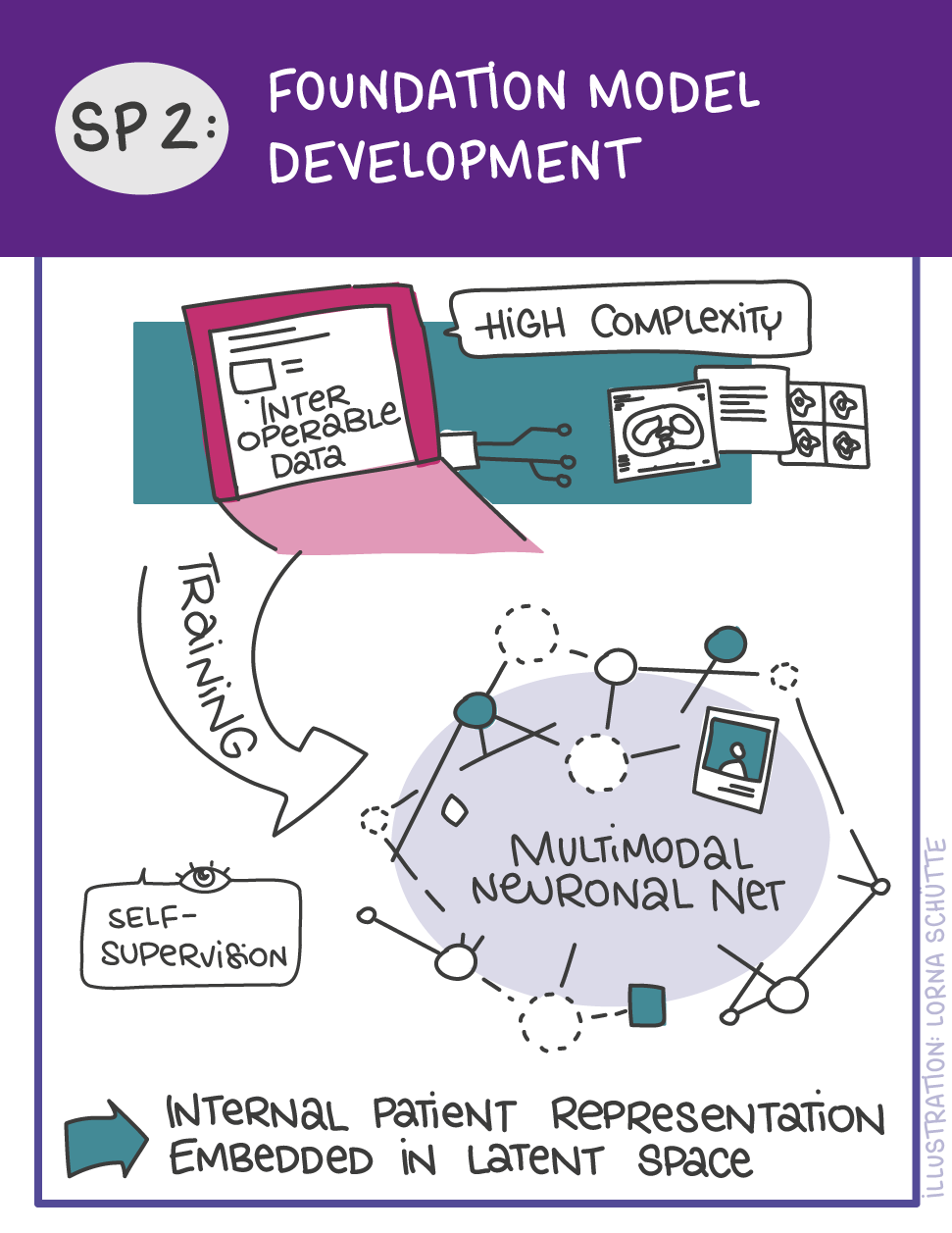
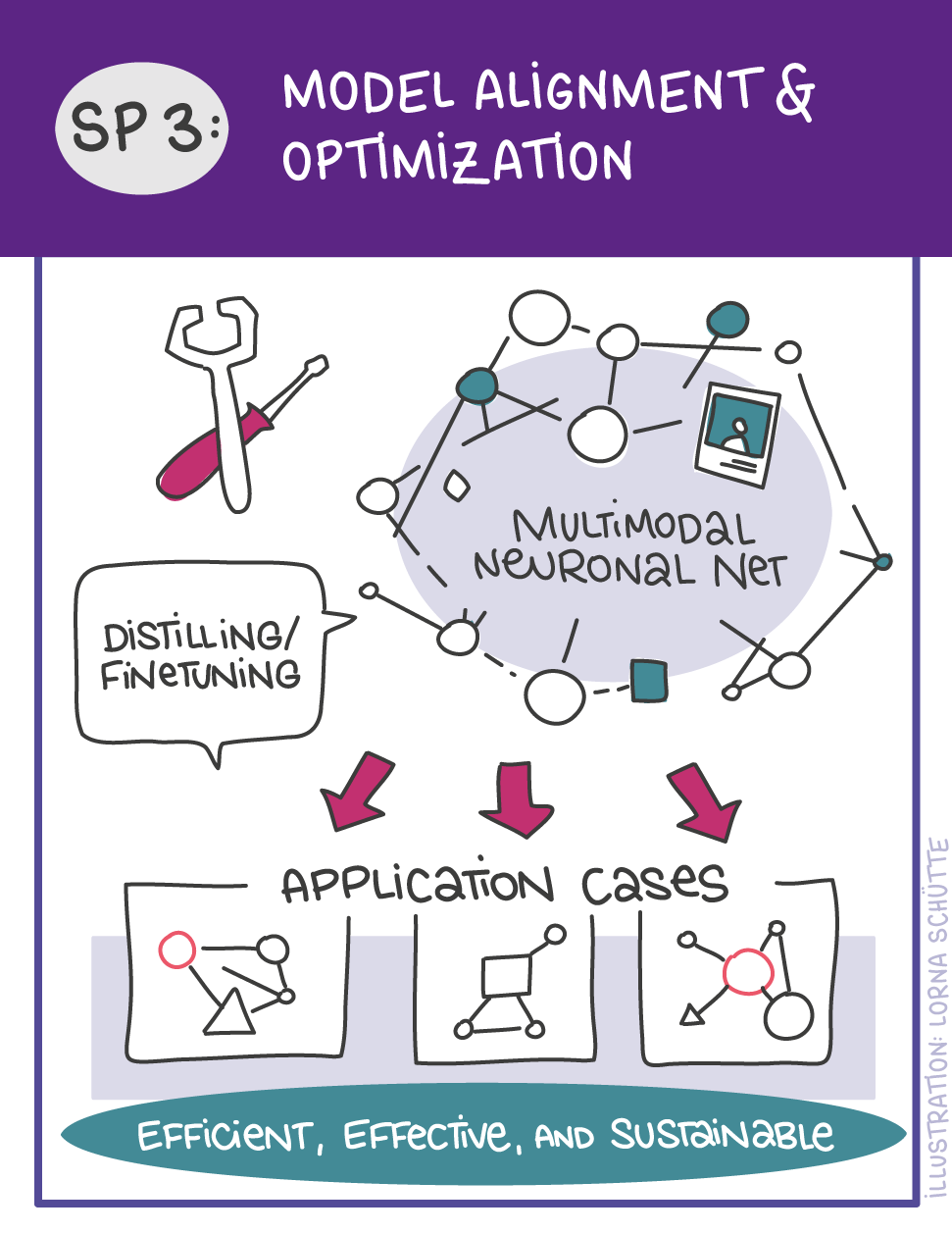
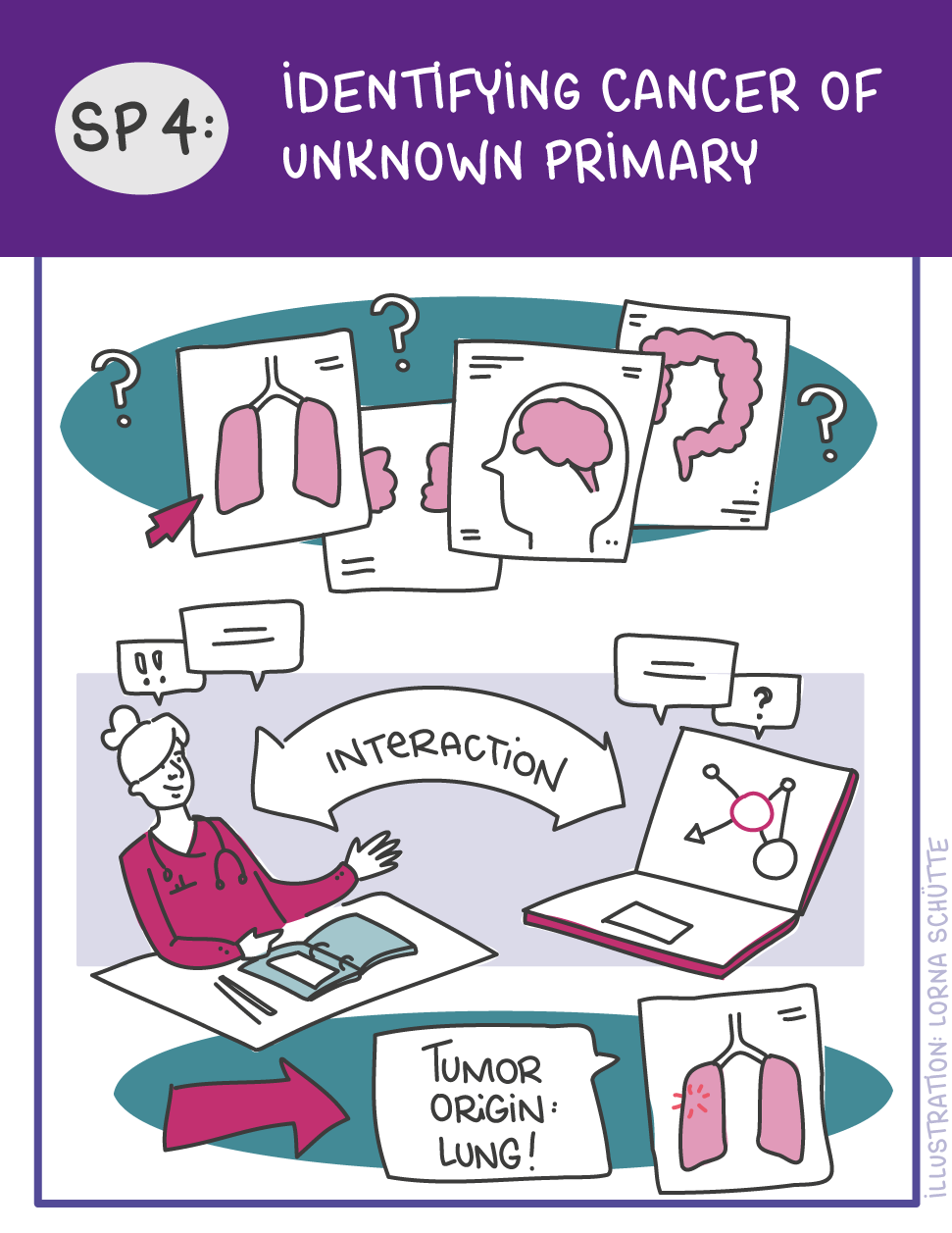
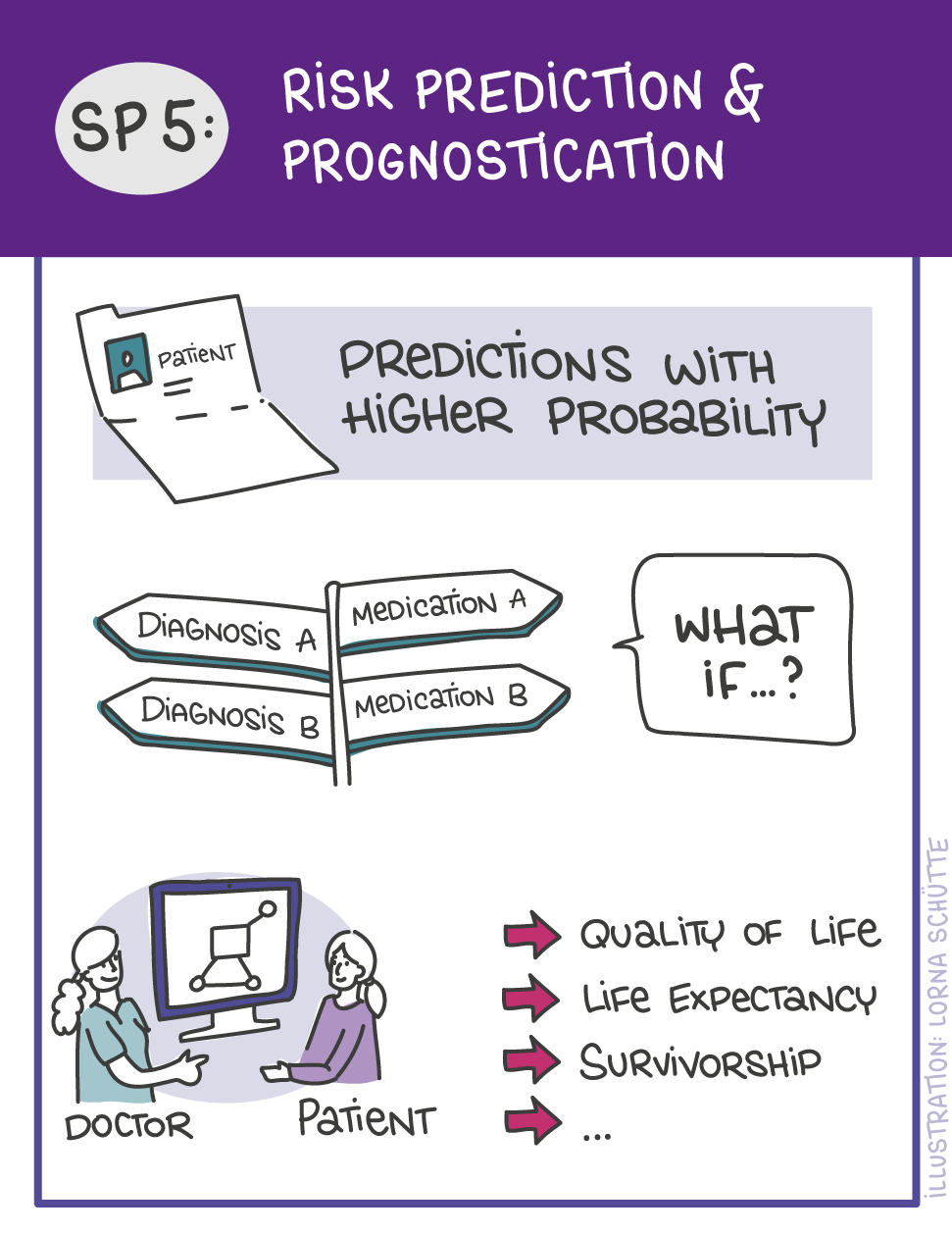
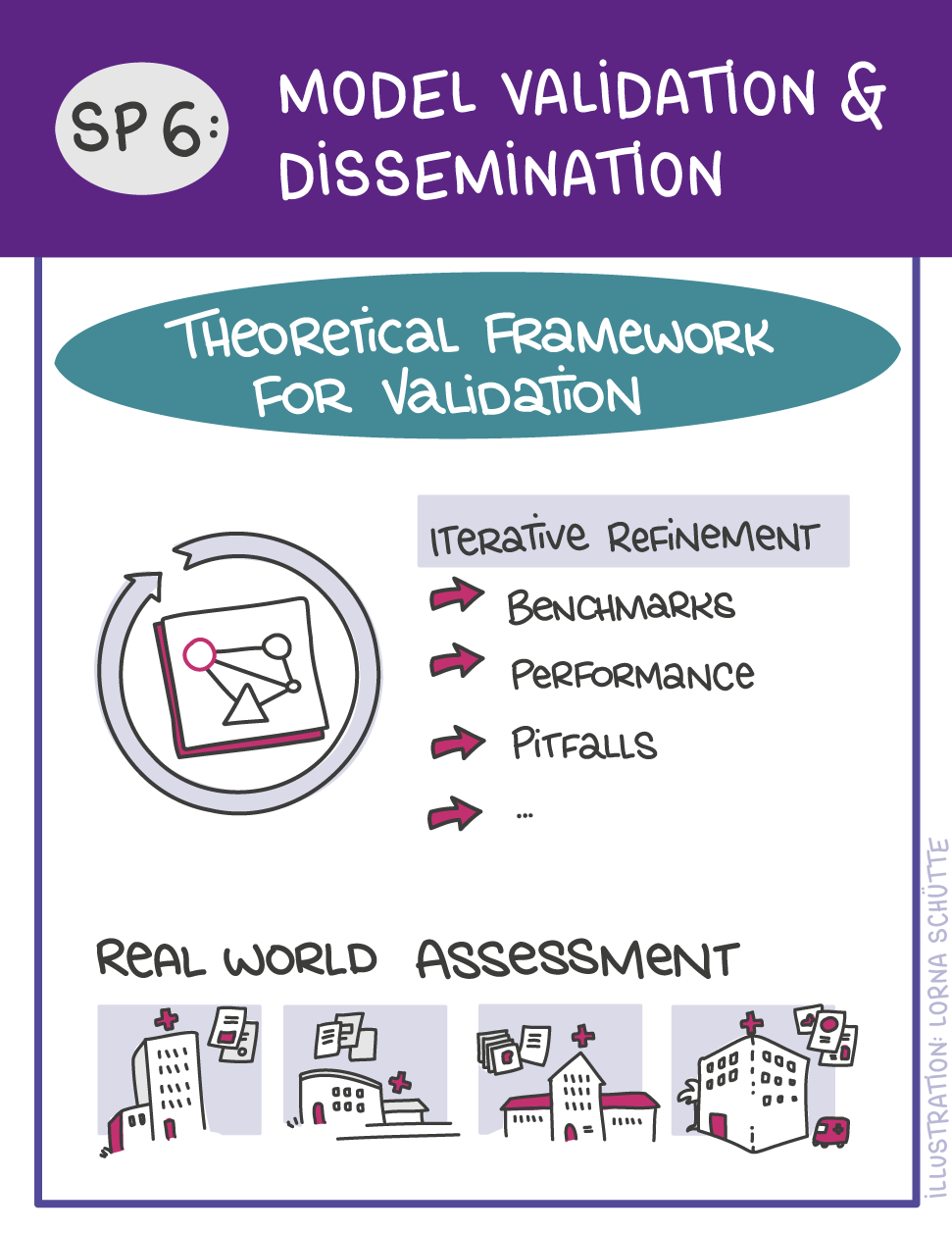
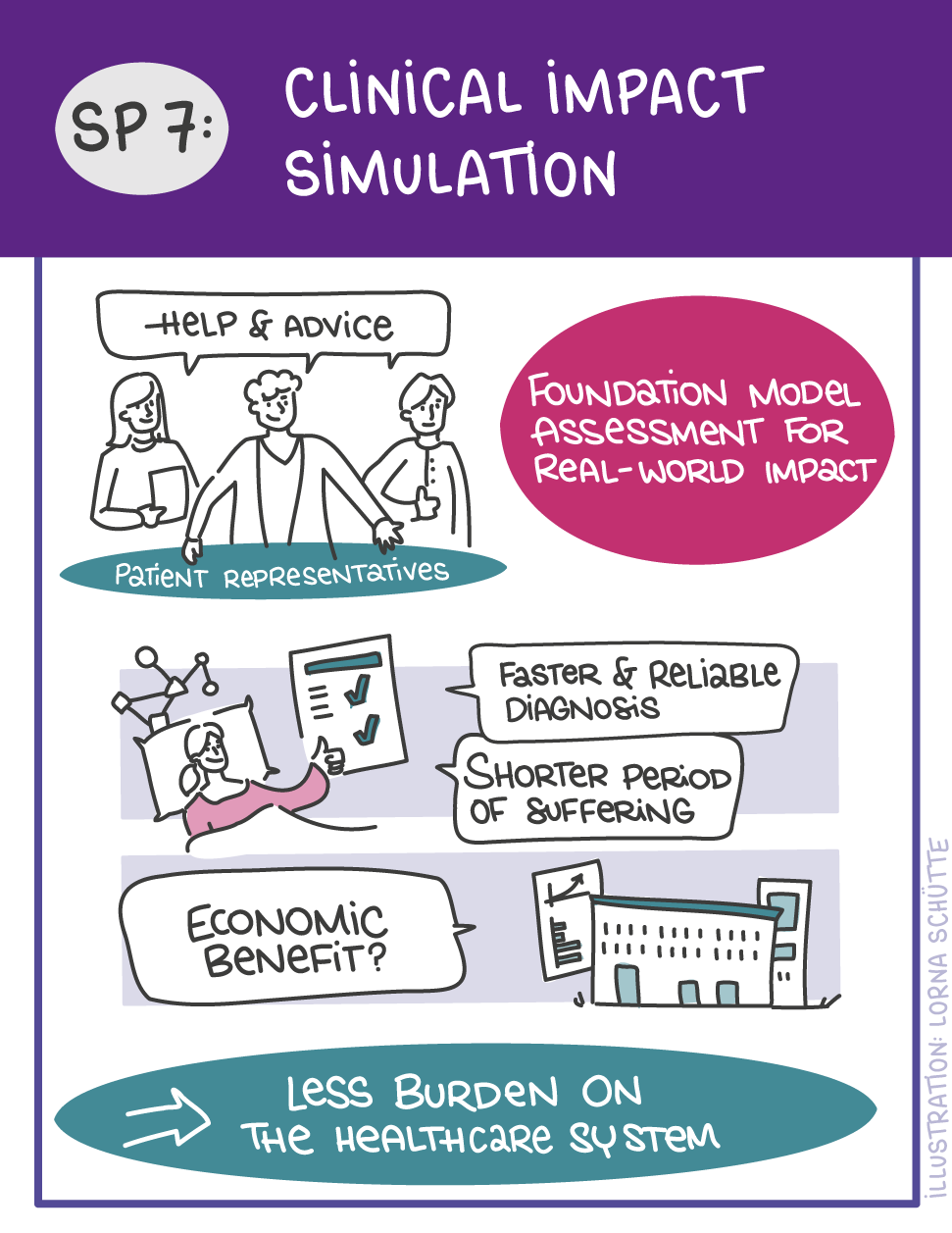
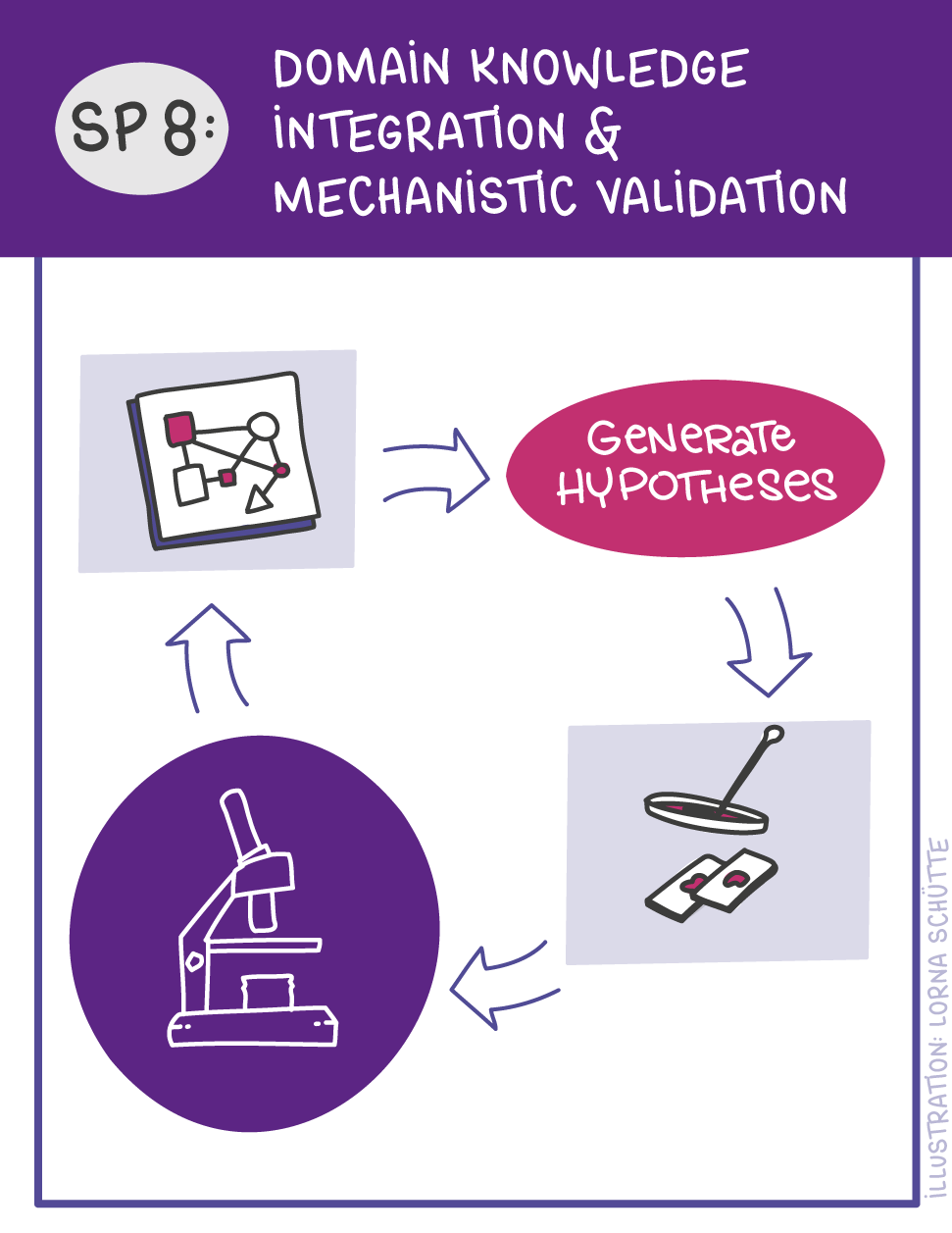
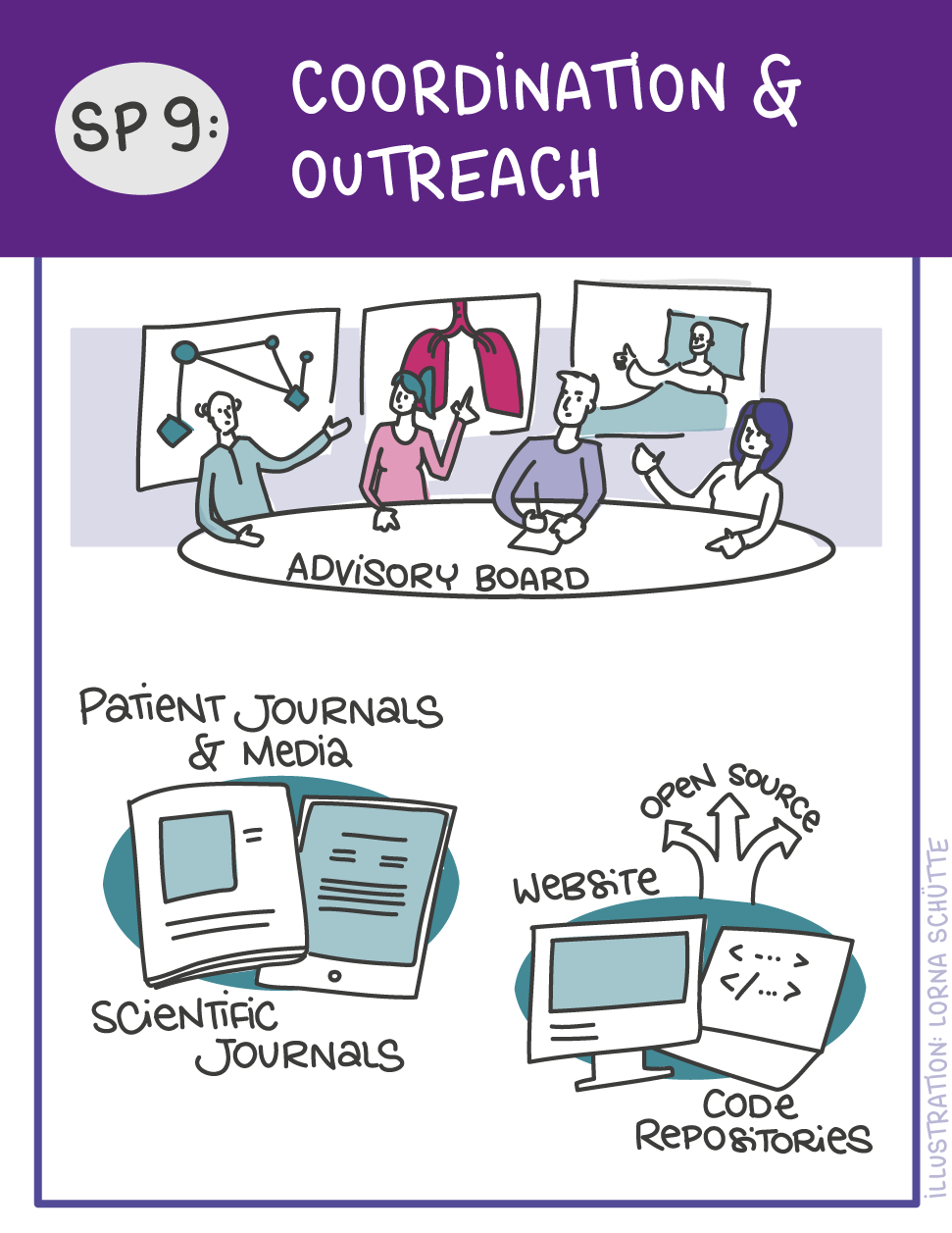
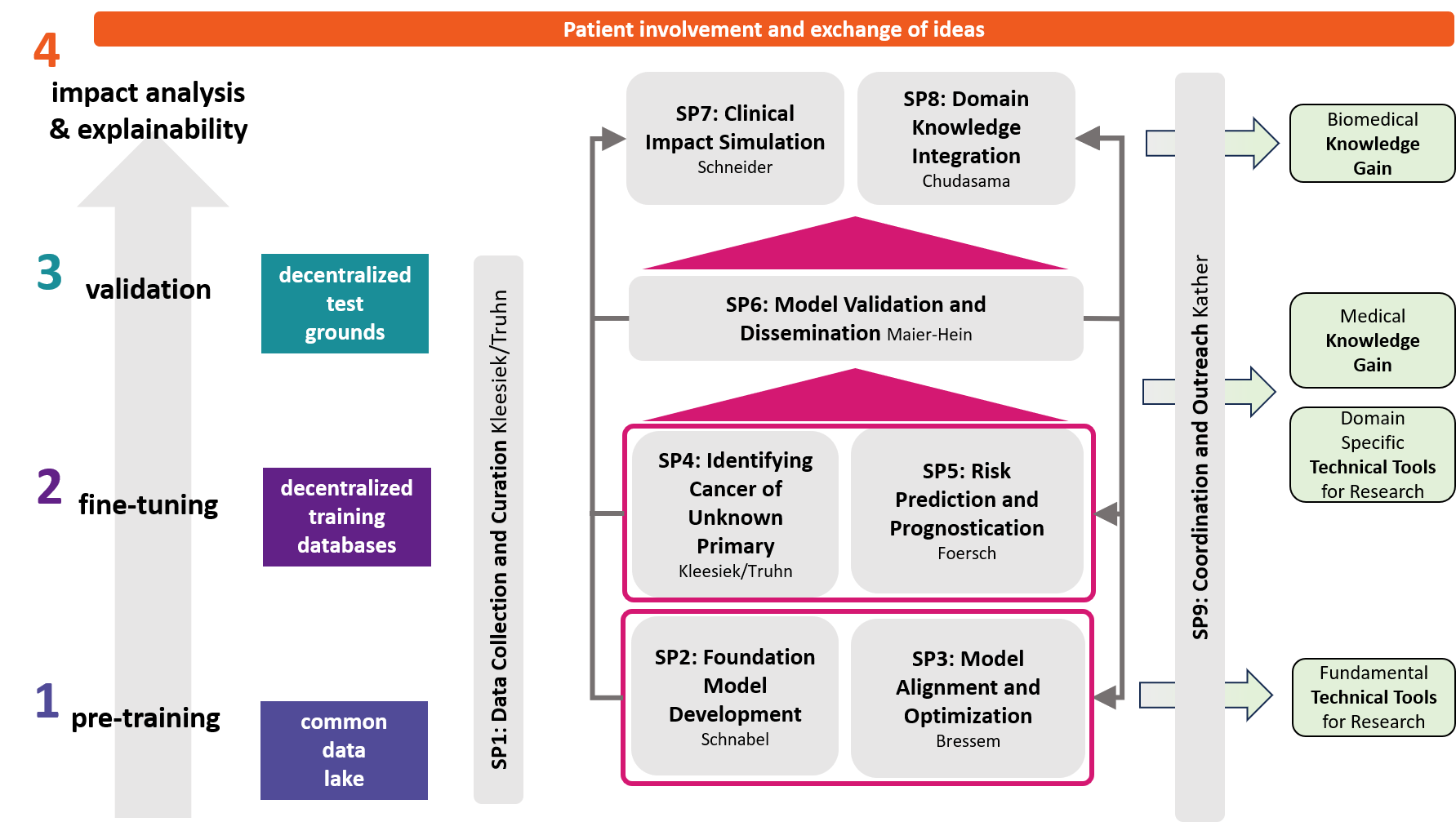
Data Management
DECIPHER-M adopts an innovative approach to data management, emphasizing the use of existing data repositories by carefully complementing them with cancer-specific well-curated data sets. The project aims to consolidate and combine data from various sources to facilitate focused research on understanding metastatic processes. This meta-data collection approach is key to avoiding redundant structures and ensuring a concentrated effort on biomedical discovery. DECIPHER-M leverages a diverse array of data resources, each serving a unique purpose in the context of AI-driven oncology research. These resources are categorized into three distinct types, ensuring a structured approach to data utilization and integration. 1) publicly available data sets, 2) proprietary data for fine-tuning, and 3) data sets of external consortia for independent validation.
The training of foundation models in sub-project 2 will make use of the collection of data in a data lake. This data lake will contain a large-scale, diverse collection of datasets from various sources. These datasets include histopathology slides, radiology images, electronic health records (EHR) data, natural language, and omics data, all of which are integral to our consortium’s interests. The objective is to use self-supervised learning to pre-train models on these datasets before fine-tuning them to specific tasks. The diversity of data sources and disease entities is crucial in this context. Self-supervised learning aims to identify general patterns, which are then applicable in supervised fine-tuning for downstream applications. The study’s principal investigators (PIs) have experience with several large-scale, public, or semi-public datasets, accessible upon request. They will contribute these datasets to the consortium. Additionally, there are plans to expand these datasets throughout the project’s duration.
- UK Biobank: A comprehensive biomedical database and research resource from the UK, containing detailed genetic and health information from half a million participants. It includes a comprehensive set of MRI scans of 60,000 individuals, making it a valuable resource for validating research findings.
- TCGA (The Cancer Genome Atlas): A project that catalogs and makes publicly available genetic mutations and histopathology images across all cancer types.
- Duke: A single-institutional, retrospective dataset of 922 invasive breast cancer patients over a decade, featuring demographic, clinical, pathology, treatment, and genomic data. It includes pre-operative dynamic contrast enhanced (DCE)-MRI images.
- NAKO: The German National cohort (NAKO Gesundheitsstudie) is Germany’s largest cohort study, involving 200,000 individuals aged 20-69 years, of whom 30,000 have received baseline MRI. Launched in October 2014 with a projected duration of 20-30 years, it aims to investigate major diseases like cardiovascular, cancer, diabetes, neurological, psychiatric, infectious, respiratory, and musculoskeletal disorders. The NAKO Health Study has collected data from 205,000 participants in its initial examinations, and this data is now available for analysis by the German scientific community through the TransferHub.
- TCIA (The Cancer Imaging Archive): A service that de-identifies and hosts a large archive of medical images of cancer, the population overlaps with TCGA.
- ‘All of us’: The US pendant to the UK biobank, with genetic and health outcome data for at least 200,000 diverse participants.
- CPTAC (Clinical Proteomic Tumor Analysis Consortium): An initiative by the National Cancer Institute to advance the understanding of cancer’s molecular basis through large-scale proteogenomic analysis. It aims to improve cancer diagnosis, treatment, and prevention by identifying proteins from altered cancer genomes and their biological processes. CPTAC has provided genomic data for over 1500 cancer patients across various cancer types to the Genomic Data Commons (GDC), including DNA and RNA sequences.
- PMBB (Penn Medicine Biobank): A dataset from the University of Pennsylvania containing health records of 60,000 patients, notable for its diversity with 30% African American participants. It includes exome wide sequencing connected with recorded health outcomes. Dr. Schneider, an adjunct professor at the University of Pennsylvania, has access to this dataset for training and validation in a diverse patient group.
- MIMIC (Medical Information Mart for Intensive Care): A large, freely-available database comprising de-identified health-related data associated with over forty thousand patients who stayed in critical care units.
- RADCURE: This dataset represents a major open-source head and neck radiation therapy data collection from the University of Toronto and Princess Margaret Cancer Centre. It includes extensive data from 4,000 patients, of which 2,745 were fully reconstructed with CT images, RT structures, and linked to existing prospectively collected outcomes data. The dataset comprises nearly 350 GB of imaging data and 14 associated clinical variables and outcomes data for each patient.
- NLST (National Lung Screening Trial): A randomized, multi-site study to assess lung cancer mortality reduction in high-risk individuals through screening. Conducted by the Lung Screening Study and American College of Radiology Imaging Network, it involved over 53,000 participants aged 55-74 with a heavy smoking history, enrolled between 2002-2004. Participants underwent three annual screenings using low-dose CT or chest X-rays from 2002–2007, with follow-up until 2009. The trial aimed to compare the effectiveness of these screening methods in reducing lung cancer mortality.
- LIDC (Lung Image Database Consortium): Initiated by the National Cancer Institute and supported by various partners, the LIDC has created a comprehensive database for the development of computer-aided diagnostic methods for lung nodule detection and assessment. The database includes 1018 thoracic CT scan cases, each paired with an XML file detailing annotations from a two-phase review by experienced radiologists. This process involved independent and collaborative evaluations of CT scans to categorize and identify lung nodules, aiming for thoroughness without requiring consensus.
- PAIP (Pathology AI Platform): A free research support platform for pathology artificial intelligence, PAIP provides a high-quality dataset for medical AI applications. The dataset, contributed by Seoul National University Hospital in South Korea, includes a variety of pathological images covering several types of cancer such as hepatocellular carcinoma, colorectal adenocarcinoma, prostatic adenocarcinoma, renal cell carcinoma, pancreatic ductal adenocarcinoma, and cholangiocarcinoma.
- MCO Study: A prospective collection from the MCO research group at University of New South Wales, encompassing clinicopathological data and tissue samples from over 1500 individuals who underwent curative resection for colorectal cancer between 1994 and 2010. It includes comprehensive clinical follow-up data for up to five years, stored in a relational database alongside key genetic data.
Supervised fine-tuning for specific medical tasks will occur at our own institutions, using proprietary datasets from our patients. The primary contribution will come from University Hospital Essen, alongside data from other institutions. We detail below the data available from day one of our project, ready for immediate use. However, we anticipate substantial efforts to greatly expand this dataset. This expansion will be in close collaboration with existing data collection initiatives. These include data integration centers, funded through the medical informatics initiatives, with which many of our study’s PIs are closely involved.
- UM Essen: The West German Cancer Center (WTZ) is part of UME. It is the largest interdisciplinary cancer center in Germany. Per year more than 14,000 unique cancer patients are treated and stored in our interoperable Smart Hospital Information Platform (SHIP). Full access to complete patient records is realised with SHIP. The total number of patient records that is already available at the start of the project exceeds 180,000 (sarcoma: 11,478; prostate cancer 12,575; colorectal cancer: 9,369; breast cancer: 13,188; liver cancer: 9,142; lung cancer: 21,190; cancer of unknown primary: 3,892).
- RWTH Aachen: The radiology department at the University Hospital Aachen has a dedicated focus on breast MRI and liver cancer. Currently, ca. 2,000 patients per year undergo an MRI examination of the breast or liver. The total number of examinations that will be made available to DECIPHER-M will exceed 15,000 in year 1 and rise to over 20,000 at the end of the project. The examinations are accompanied by a comprehensive workup of the patients’ history (textual data), medication and risk factors (tabular data). All of these data items will be used to fine-tune the cancer specific models of step 2.
- UM Mainz: The Institute of Pathology of the University Medical Center Mainz is the only academic pathological institute in the state of Rhineland-Palatinate and has a caseload of over 50,000 cases per year resulting in around 250,000 individual histopathological slides. It is part of the University Cancer Center (Universitäres Centrum für Tumorerkrankungen UCT) with 14 certified organ-specific “sub” centers. Per year ca. 1300 breast cancer cases, 1000 colorectal cancer cases, 700 prostate cancer cases, 600 lung cancer cases, 150 liver cancer cases, and 100 sarcoma cases are seen at the Institute of Pathology in Mainz. These include the complete routine pathological spectrum from biopsy specimens for primary diagnosis to surgical resections of advanced / metastatic disease. Combined with retrospective cohorts, data of up to 5000 cases could be made available from day 1 adding another 5000 cases throughout the project. The data include digitalized histopathological and immunohistochemical slides (WSI) and pathology reports (textual data), but also molecular (NGS) and survival data (for some cases).
- TU Munich: The radiology department of the “Klinikum rechts der Isar” of the Technical University Munich (TUM) is a center for gastrointestinal tumors. Currently, 200 patients with colorectal cancer, 300 patients with pancreatic cancer and 200 patients with other gastrointestinal cancers (liver, stomach, small intestine) are treated each year and undergo imaging. Additionally TUM also treats patients with lung cancer (200/year) and other rare forms of cancer, which could be contributed to the project. Digital PACS has been available at TUM since 2006, making data of over 10 000 individual patients available at day one. Over the course of the project this will increase to 12 000. The data includes CT, MRI and ultrasound images as well as the patient’s history (textual data), which will be used to fine-tune the cancer specific models of step 2.
We aim to validate our models in large-scale external consortia in Germany and beyond. For all of these validation datasets, our study PIs are either closely involved and have worked with the data before (Kleesiek/Kather/Truhn — RACOON, Truhn/Kather — ODELIA, Kather – TANGERINE, Bressem – COMFORT) or have already established links with the consortium / data source (Kleesiek — SATURN-3) or a clear plan exists to formally request access as soon as the data becomes available for request (BfArM-FDZ).
- Bundesinstitut für Arzneimittel und Medizinprodukte (BfArM) – FDZ (Forschungsdatenzentrum): The FDZ enables access to billing data for all individuals insured under the statutory health insurance system in Germany, contributing significantly to health research. Its goal is to make these data usable for research purposes, thereby supporting improved healthcare services. Currently, the FDZ is in the process of being established and will be developed in phases. Data requests are anticipated to be possible around the third or fourth quarter of 2024.
- RACOON: An infrastructure within the National University Medicine (NUM) network. It allows scaling by including additional partners for data contribution and model dissemination. RACOON features GPU-servers with a unified software stack deployed to every university hospital in Germany. This includes the Joint Imaging Platform (JIP), an open-source platform for deploying containerized applications. Additionally, a Web-API at IKIM, University Hospital Essen, is offered for accessing foundation-models within DECIPHER-M through the Open Medical Inference framework (OMI), coordinated from Essen with Co-PI Jens Kleesiek, especially beneficial for clients lacking GPU resources.
- ODELIA: The Horizon Europe-project ODELIA builds the first pan-European swarm learning (SL) network that allows for privacy conserving training of medical AI algorithms with true democratic participation of all partners. In ODELIA, an SL algorithm will be developed, which enables multiple partners to jointly train AI algorithms without sharing any data. The AI models are trained decentrally and models are combined without the requirement for a central coordinator. Additionally, an online viewer will be used which allows anyone to apply swarm-learning-trained AI algorithms on their own data offering promising possibilities for DECIPHER-M. A central use-case of ODELIA is breast cancer. Core European breast cancer centres are involved within this project and contribute data to the SL network. This data will serve as a validation set for the breast cancer specific model.
- TANGERINE is a EU-funded consortium collecting data from patients who received ICIs for any type of cancer. The data includes histopathology slides, CT scans, and clinical outcomes, which are anonymized and analyzed by an AI system. The goal is to predict and explain ICI response, survival, and toxicity based on the data. The project aims to collect data from 2400 patients in total, with 1800 from retrospective (2017-21) and 600 from prospective (2022-24) cohorts.
- SMI: The Scottish Medical Imaging (SMI) Archive is a collection of population-based, medical radiology images from real patient records for use in health care research and the validation of artificial intelligence algorithms within the Scottish National Safe Haven. The images are linked to other routinely collected electronic health care records (such as hospital, prescribing, birth, and death data).
- COMFORT: The Horizon Europe-project COMFORT aims to develop computational models for patient stratification in urologic cancers, particularly prostate cancer (PCa) and kidney cancer (KC). The project focuses on leveraging multimodal AI, including radiological imaging like multiparametric MRI and CT scans, along with individual health data and biomarkers. It seeks to overcome current clinical limitations in diagnosing and treating PCa and KC by utilizing AI to uncover patterns in complex data sets, which are not easily discernible to medical professionals. COMFORT’s ultimate goal is to improve clinical prognosis, patient stratification, and individualized treatment for patients with PCa or KC through the development and international interdisciplinary validation of data-driven decision support systems.
- SATURN-3: A research consortium focused on the spatial and temporal resolution of intratumoral heterogeneity (ITH) in three challenging cancer types: breast, colorectal, and pancreatic cancer. SATURN-3 investigates how tumors change individually over the course of the disease, impacting treatment efficacy. The consortium’s multidisciplinary approach involves analyzing biomaterial from cancer patients before and after therapy, relapse, and metastasis. This study aims to understand ITH over time and across disease stages to develop new diagnostics and comprehensive treatment concepts. The initiative is part of the National Decade Against Cancer, supported by the German Federal Ministry of Education and Research (BMBF), promoting powerful, internationally competitive alliances to improve cancer treatment.
Compute Ressources
To make use of the data and train the models, considerable compute resources are needed. Several of the PIs have access to dedicated compute clusters due to their background in AI model development and research. The necessary computing power to conduct DECIPHER-M will be provided as in-kind contributions by the partners. In detail, the following GPU-clusters are available and have been agreed upon to be available for training of the models:
Site | Available Compute at Start of Project | Available Compute in Y2 |
---|---|---|
Aachen | 204 x NVIDIA H100 | 500 x NVIDIA H100 (or equivalent) |
CLAIX-2023 consists of over 660 compute nodes with 2x Intel Sapphire Rapids processors, each with 48 cores and 256 to 1024 GB DDR5 memory. In addition, there are 51 compute nodes of identical basic architecture, each equipped with four NVIDIA Hopper H100 GPUs (incl. NVLink) as accelerators. For interactive work with the system, CLAIX-2023 also has several dialogue systems with compatible architecture, offering a jupyter-based access (among other services). All nodes are connected to an NVIDIA/Mellanox NDR InfiniBand 200 Gigabit/s network and several filesystems. As a national HPC resource in Germany, CLAIX-2023 allows to execute large-scale HPC and AI applications at high efficiency. Access to CLAIX resources comes with methodological service and support, ranging from first-level user support to consulting and analysis services on performance and scalability of HPC and AI workflows. Access is granted with a dedicated support for DECIPHER-M and its multi-year collaborative research. CLAIX-2023 will be complemented by the next system expected to start operation in Q4/2025. |
||
Essen | 66 x NVIDIA A6000, 56 x NVIDIA A100, 40 x NVIDIA H100, 2 x NVIDIA GH200 | |
At IKIM/UME, we have deployed two specialized cluster domains tailored for the research with multimodal medical data: one domain outside of the hospital infrastructure for training models on anonymized data (IKIM-extern) and a second cluster domain inside the hospital network for training models with not fully anonymised/-able data such as DNA (KI-Translation Essen as part of the REACT-EU initiative with € 2.75M hardware budget, EFRE-0801977). All servers are installed in the UME data centers, which fulfill the KRITIS requirements. Most of the data for research are stored in Fast Healthcare Interoperable Resource (FHIR) and in Digital Imaging and Communications in Medicine Picture Archiving and Communication System(DICOM PACS) to ensure standardized, reproducible data pipelines for model training and inferencing. The IKIM-extern infrastructure includes 11 GPU training nodes equipped with 6x NVIDIA A6000 GPU and 2 TB RAM each, 2 GPU nodes with 8x NVIDIA DGX A100, 2 GPU inference nodes, and 145 CPU nodes (4,630 CPU cores and 27.8 TB RAM) and mirrored ZFS HDD storage (nearly 2 PB usable capacity). KI-Translation Essen infrastructure consists of 10 GPU Servers, each equipped with 4x NVIDIA A100 80GB and 2 TB RAM, 1 NVIDIA DGX H100, 10 hyper-converged CPU Servers, each with 64 Cores, 1 TB RAM, 64 TB NVMe SSD, and an all-flash distributed storage appliance with nearly 800 TB usable capacity and > 300k achievable IOPS. The GPU capacity is currently being upgraded with 4 additional GPU nodes, each with 8x H100 80 GB SXM5 GPU and 2 GPU nodes with NVIDIA GH200 96 GB Grace Hopper. The new nodes will be connected with 400 Gigabit/s Infiniband DirectGPU networking and will be available for the project in Q2/2024. |
||
Dresden | 39 AMD Rome nodes, each 8 Nvidia A100 GPUs, 32 IBM Power 9 nodes, each 6 Nvidia V100 GPUs, 192 AMD Rome nodes, each 128 cores, 512 GB RAM with 400 GB/s bandwidth, 2 PB fast flash memory (NVMe), 10 PB archive with access via S3, Cinder, NFS, QXFS | Planned 100 Nvidia H100 and 100 Nvidia A100 GPUs in addition to the baseline |
TU Dresden offers a high performance computing (HPC) facility located at the university’s center for information services and high performance computing (ZIH). ZIH operates high performance computing systems with almost 100.000 processor cores, more than 500 GPUs, and a flexible storage hierarchy with more than 40 PB total capacity. The HPC systems provide an optimal research environment especially in the area of data analytics and machine learning as well as for processing extremely large data sets. Moreover it is also a perfect platform for highly scalable, data-intensive and compute-intensive applications. In particular, for High Performance Computing / Data Analytics (HPC-DA) different technologies are combined to individual and efficient research infrastructures. Especially for applications in the area of machine learning and deep learning 192 powerful Nvidia V100 GPUs are installed. Resources can be used also interactively, for example with Jupyter Notebooks. For data analytics on CPUs a cluster with high memory bandwidth is provided. To efficiently access large data sets 2 petabytes of flash memory with a total bandwidth of about 2 terabytes/s are available. Additionally, 312 Nvidia A100 GPUs are provided especially for machine learning applications. All of this computer infrastructure is professionally managed by dedicated teams and adheres to the high privacy standards which govern data processing and exchange in the European Union, including the General Data Protection Regulation (GDPR). | ||
Heidelberg | 96 x NVIDIA V100, 96 x NVIDIA A100, 48 x TitanRTX, 4 x RTX6000, 2 x NVIDIA Grace Hopper | Substantial upgrade planned |
The main AI infrastructure at DKFZ is an IBM Spectrum LSF-based GPU Cluster for model training, consisting of 64 nodes within our data center with configurations ranging from 24 to 128 CPU cores and from 188 GB RAM to around 1.5TB RAM equipped with GPUs varying from NVIDIA RTX 2080Ti to NVIDIA A100. Seven of these nodes are based on NVIDIA DGX systems utilizing either 8 x V100 16GB (1 node), 16 x V100 32GB (2 nodes) or 8 x A100 40GB (4 nodes). | ||
Munich | 86 x NVIDIA V100, 114 x NVIDIAA100 | Substantial upgrade planned |
The main AI infrastructure at Helmholtz Munich consists of currently 200 GPUs (V100s and A100s). Several nodes are based on NVIDIA DGX systems utilizing either 16 x V100 32GB (2 nodes), 8 x A100 40GB (3 nodes) and 8 x A100 80GB (1 node). They are complemented by 48 x A100 80GB and 50 x A100 40 GB and more V100s. Additionally, there are 18,672 CPUs (Intel and AMD) available. Storage comprises a DD Exascale Lustre File System with 18+ PB and a super-fast 0.5+ PB DD NVME. There are up to 1.9 TB storage per computer node. | ||
Mainz | 1 x NVIDIA DGX WS 1. Gen. 5 x NVIDIA RTX3090 | Application for MOGON HPC planned |
The AI infrastructure of the Institute of Pathology consists of one NVIDIA DGX WS (1. Gen) and several RTX3090 for smaller individual experiments. However, researchers at the Johannes Gutenberg University Mainz can apply for access to the MOGON HPC. This comprises 590 compute nodes, each equipped with dual AMD EPYC 7713 processors. These processors have 64 cores, a 2 GHz clock speed, and 256 MB of L3 cache. The cluster has a total of 75,000 CPU cores and includes 40 A100 Tensor core GPUs for advanced computational tasks. It offers 186TB of RAM and a significant fileserver storage capacity of 8,000 TB. The overall peak performance of the MOGON NHR Süd-West Cluster is 2.8 PetaFLOPS. |
Patient Survey:
“Use of AI tools to better predict, detect and treat metastasized cancer”
Patient involvement is central pillar for our proposal. In order to incorporate patients’ expertise and perspectives into our research design from its very inception, together with the participating POs, we created a survey investigating different aspects of metastasis affecting the patients. These included, but were not limited to disease characteristics, clinical experience as a cancer patient, social and emotional aspects including quality of life while living with fear or experience of metastatic disease. Importantly, we also queried the current standpoint on use of artificial intelligence in clinical decision-making.
The survey was disseminated by all participating patient organizations (POs) in Germany and the Netherlands via mailing lists and social media, as well as through professional networks of participating investigators of the consortium. Within a short span of two weeks, a staggering amount of responses were received (n=650) from patients affected by diverse cancer types covering all age groups from adolescent and young adults to elderly patients. The willingness to participate and give a detailed description of personal experience wherever possible highlighted enthusiasm as well as unmet needs of the patients.
The results of the survey highlighted following key aspects:
- Strength of outreach capacities of our partner patient organizations.
- High metastasis burden in common and rare cancer entities, including survey responders experiencing metastatic lesions in hundreds and large lesions at the time of diagnosis.
- About 50% of responders were sarcoma patients, underlining unmet medical need in this large and heterogeneous group of rare cancers.
- Profoundly negative experience around clinical monitoring of the disease, mainly associated with uncertainty of diagnosis, long waiting times, treatment side effects, delayed detection of metastasis in spite of multiple visit to the clinics and absence of specialized care
- Significantly impaired quality of life due to fear of relapse and metastasis, especially before clinical imaging scans.
- A thoroughly positive viewpoint on implementation of AI-assisted clinical care and willingness to share own comprehensive clinical data for this purpose.
- Skepticism around AI-assisted clinical care in a minority of patients attributed to missing information, highlighting the need for timely education and dissemination of these approaches for effective clinical implementation.
Multiple aspects of the survey results were immediately actionable in the building of the proposal and have been implemented and mentioned accordingly. As elaborated in the proposal, we will involve patients as true partners of our research, and work with them to leverage the newest generation of AI-based foundation models and biological validation to push improved detection, risk prediction and tailored treatment strategies for cancer patients.
Detailed results of the survey are available for download here.