Deciphering Metastasis with
Multimodal Artificial Intelligence Foundation Models
DECIPHER-M
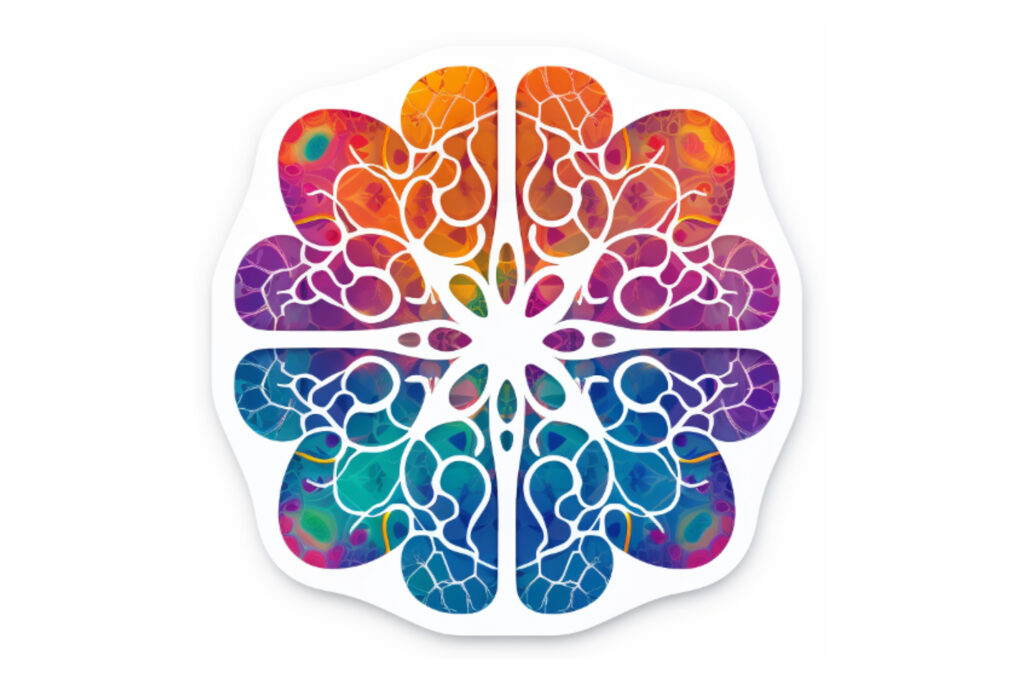
About DECIPHER-M
Metastasis is the cause of death for most cancer patients. However, the understanding of its underlying mechanisms is very incomplete.
DECIPHER-M addresses this with a unique approach using a new form of artificial intelligence (AI) called multimodal foundation models. In this project, these models are used to analyze a wide range of data, such as radiological images, pathological reports and genetic information of a patient together. This will answer fundamental questions about metastasis, such as the mechanisms of its occurrence, the potential to predict who might develop it and where, and what type of treatment might be most effective for different patients.
In addition, DECIPHER-M will provide practical tools that can be applied to individual patients to customize screening and treatment in cases at high risk of metastasis. Specifically, these tools aim to predict the most effective treatment for individual patients with metastatic disease so that these patients can be treated more effectively.
Facts and figures
Coordinator: TU Dresden
Number of Partners: 7
Start Date: March 1, 2025
End Date: February 29, 2028 (with potential extension)
Total Funding: around € 5.5 million (additional €3.5 million upon extension)
This project has received funding from the German Federal Ministry of Education under grant agreement No. 01KD2420A.
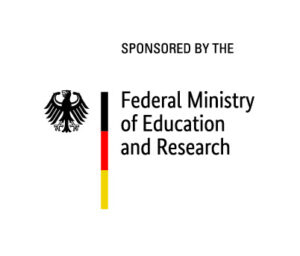
Views and opinions expressed are however those of the author(s) only and do not necessarily reflect those of the BMBF and the granting authority can’t be held responsible for them.
Project Plan
The objectives of DECIPHER-M are addressed with a strategic work plan, which is divided into 9 subprojects (SP).
First, data will be collected in SP1. In SP2, this data will be used and basic machine learning models will be developed that can use a variety of medical inputs (images, text, tabular data, etc.). The code and methodology to fine-tune these foundation models based on real-world clinical datasets will be developed in SP3. This will enable the models to learn specific medical knowledge and gain a comprehensive understanding of the relationships between image and non-image data. This code base will then be used to develop specialized, fine-tuned models for specific use cases. In particular, the focus is on two clinical scenarios that are of great importance: Identification of cancer of unknown cause in SP4 and risk prediction and prognosis in SP5. These advanced models will be validated in SP6, which deals with rigorous model evaluation. Once the models have been validated in this way, the clinical impact will be investigated in real clinical simulation studies in SP7. Based on these findings, the application scenarios of the models in SP4 and SP5 will be adapted to the needs of patients. Building on the previous sub-projects, SP8 is dedicated to translation into mechanistic research to potentially uncover new molecular changes with potential implications for treatment. Throughout the project, there will be close cooperation with patients and the collaboration of the consortium will be organized in SP9.
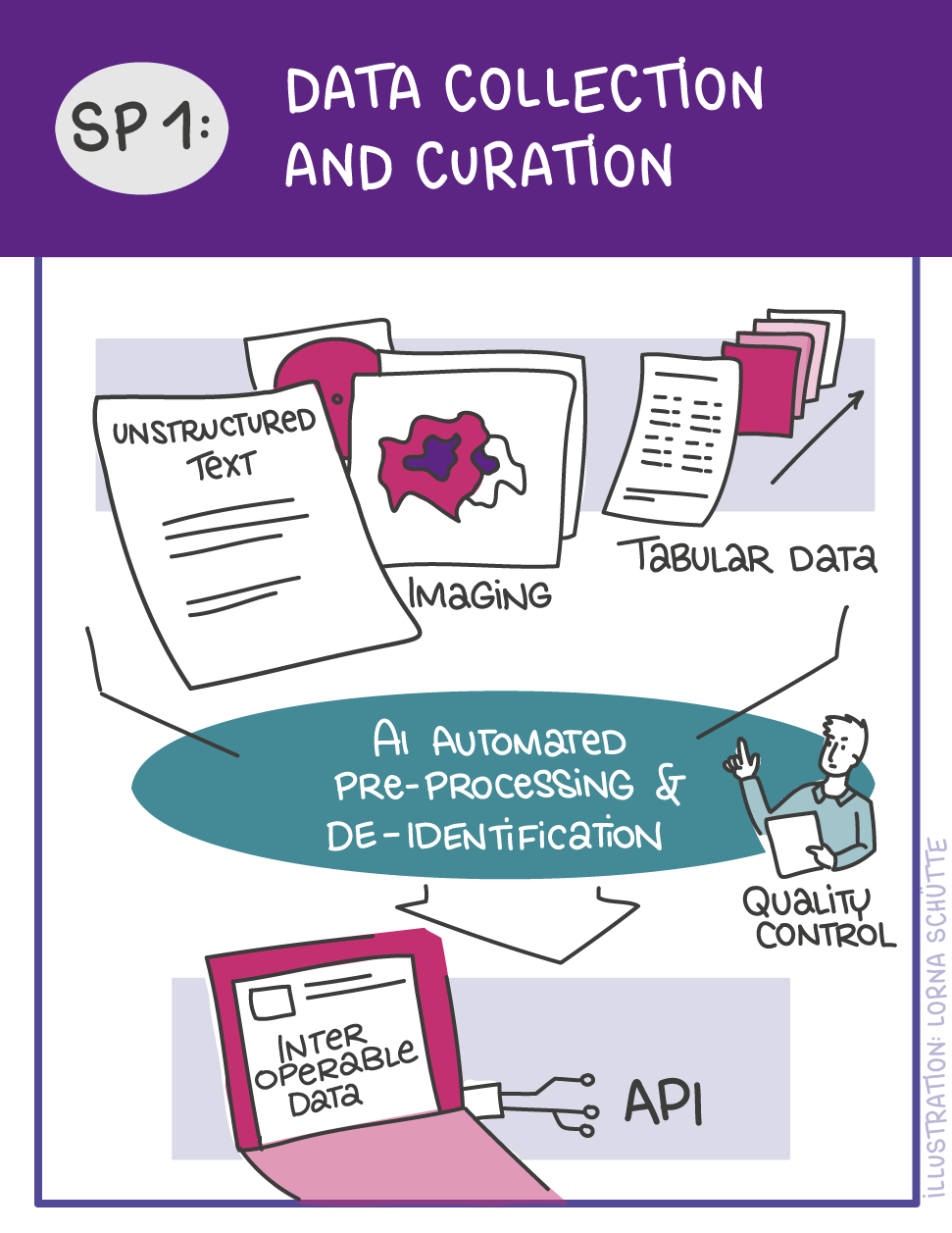
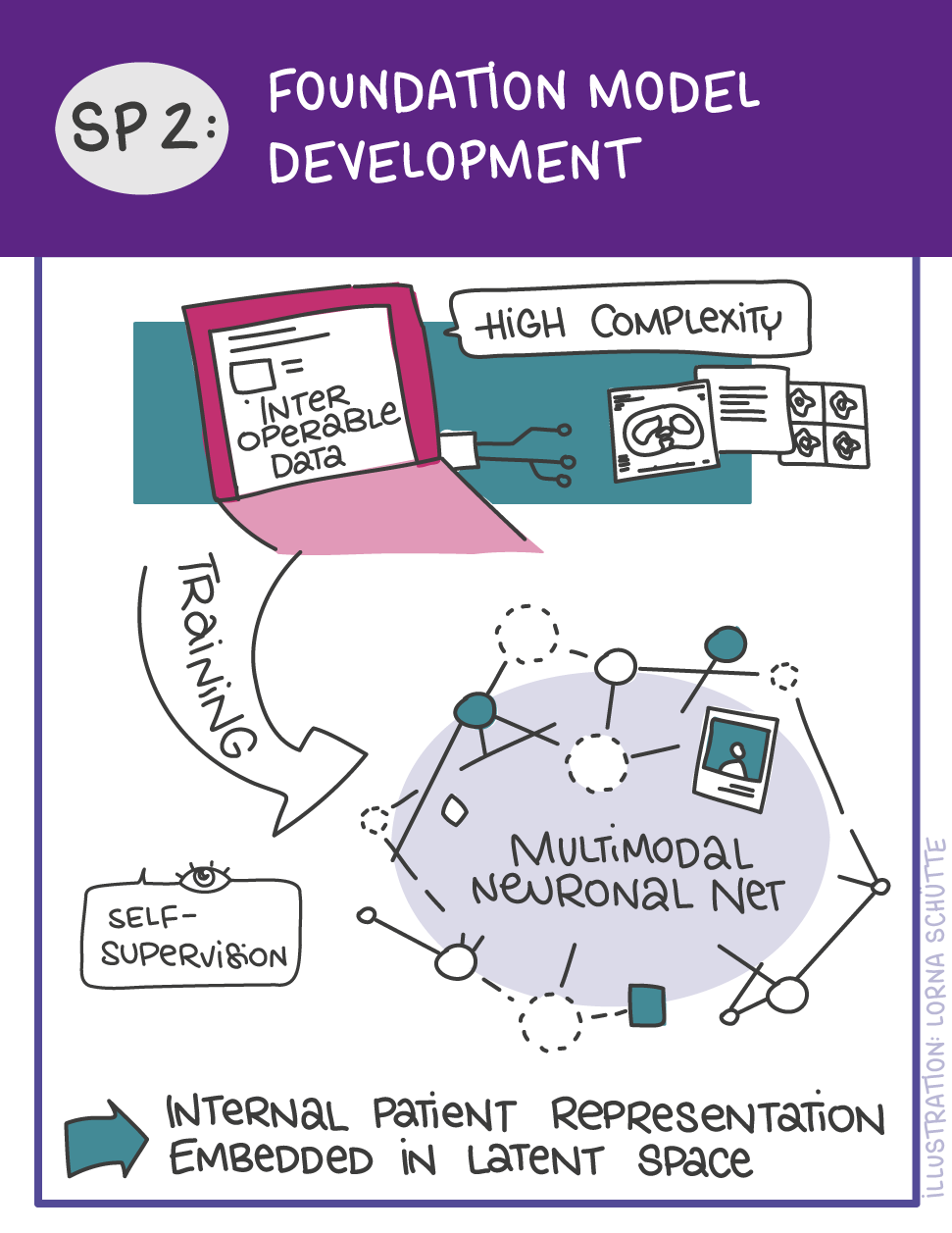
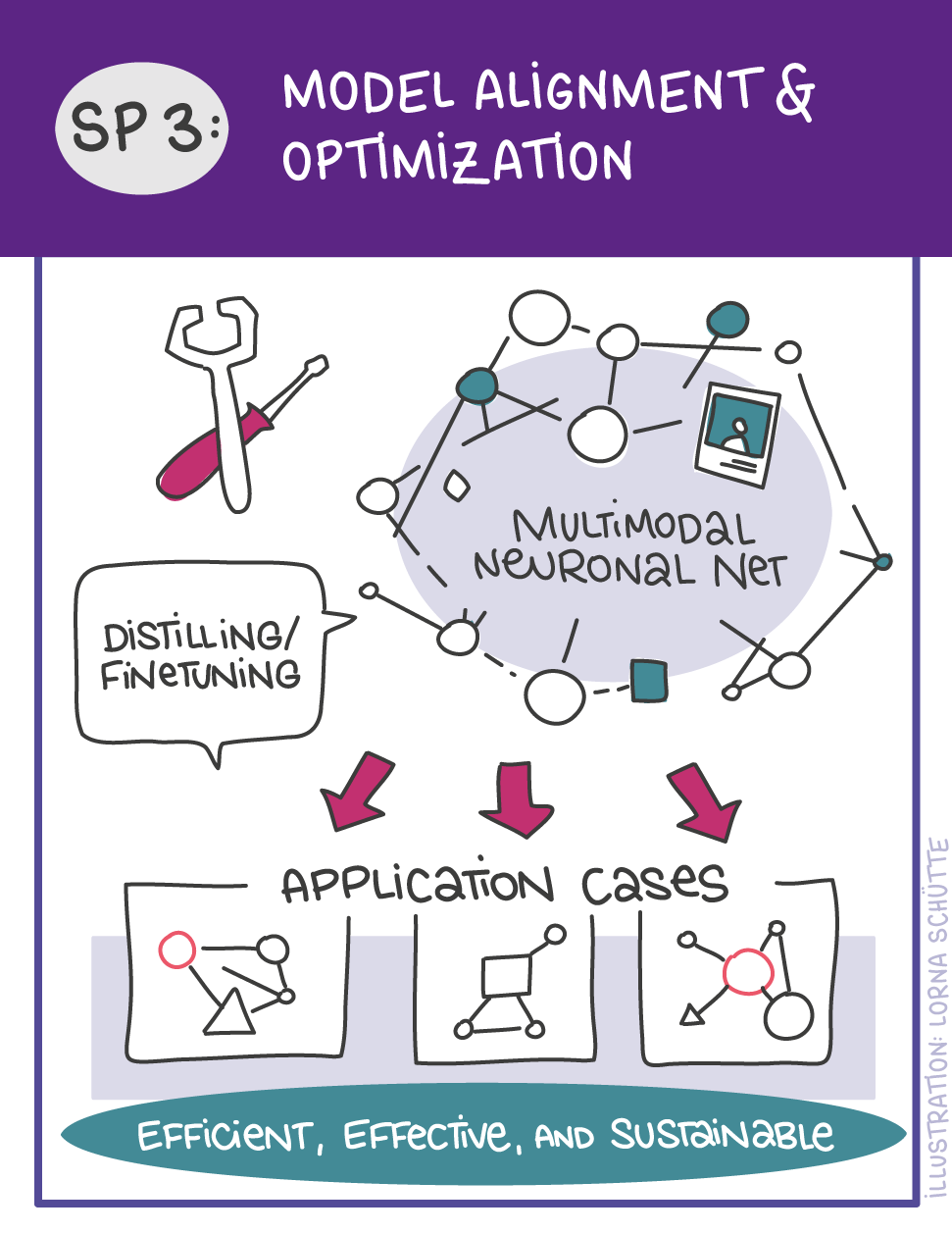
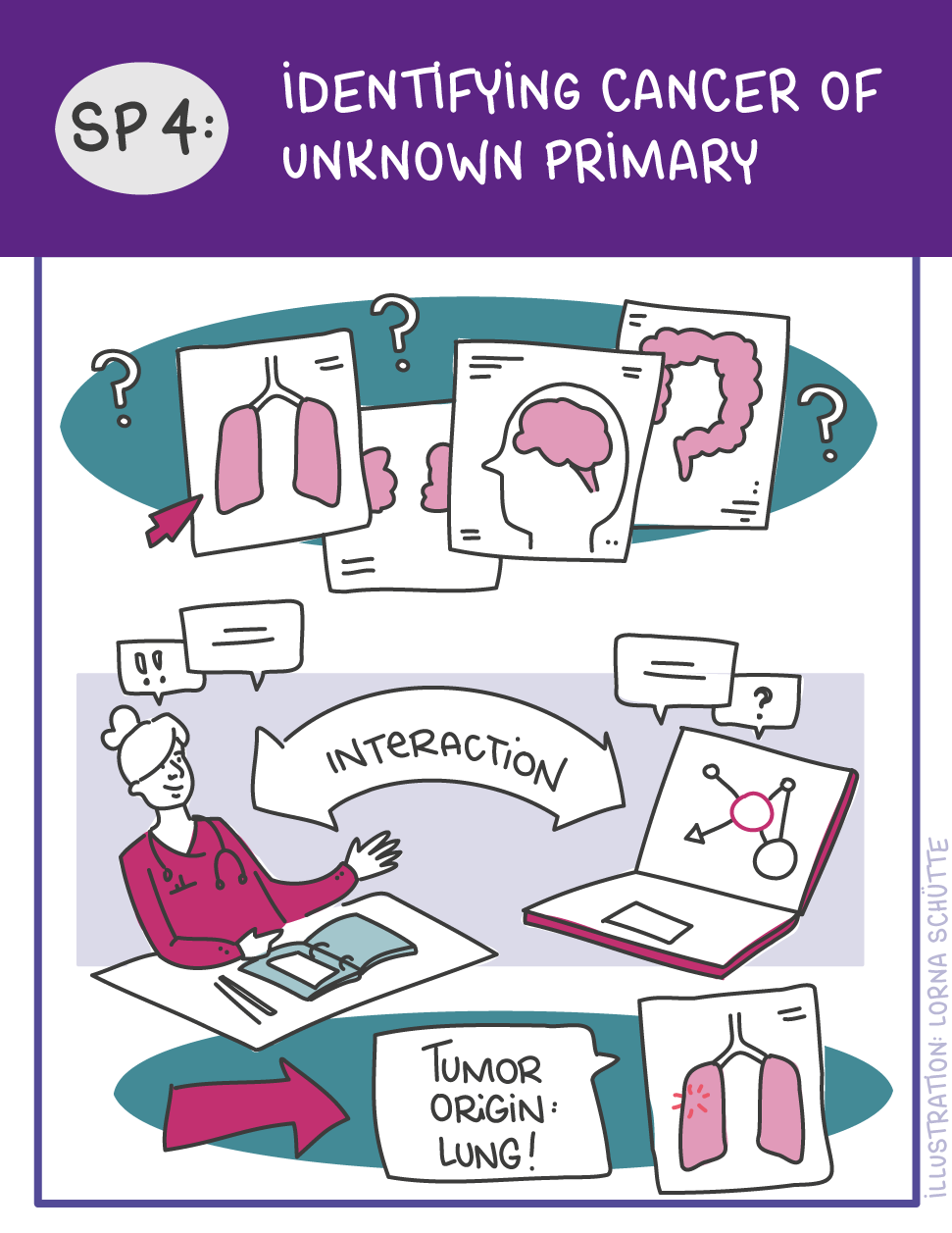
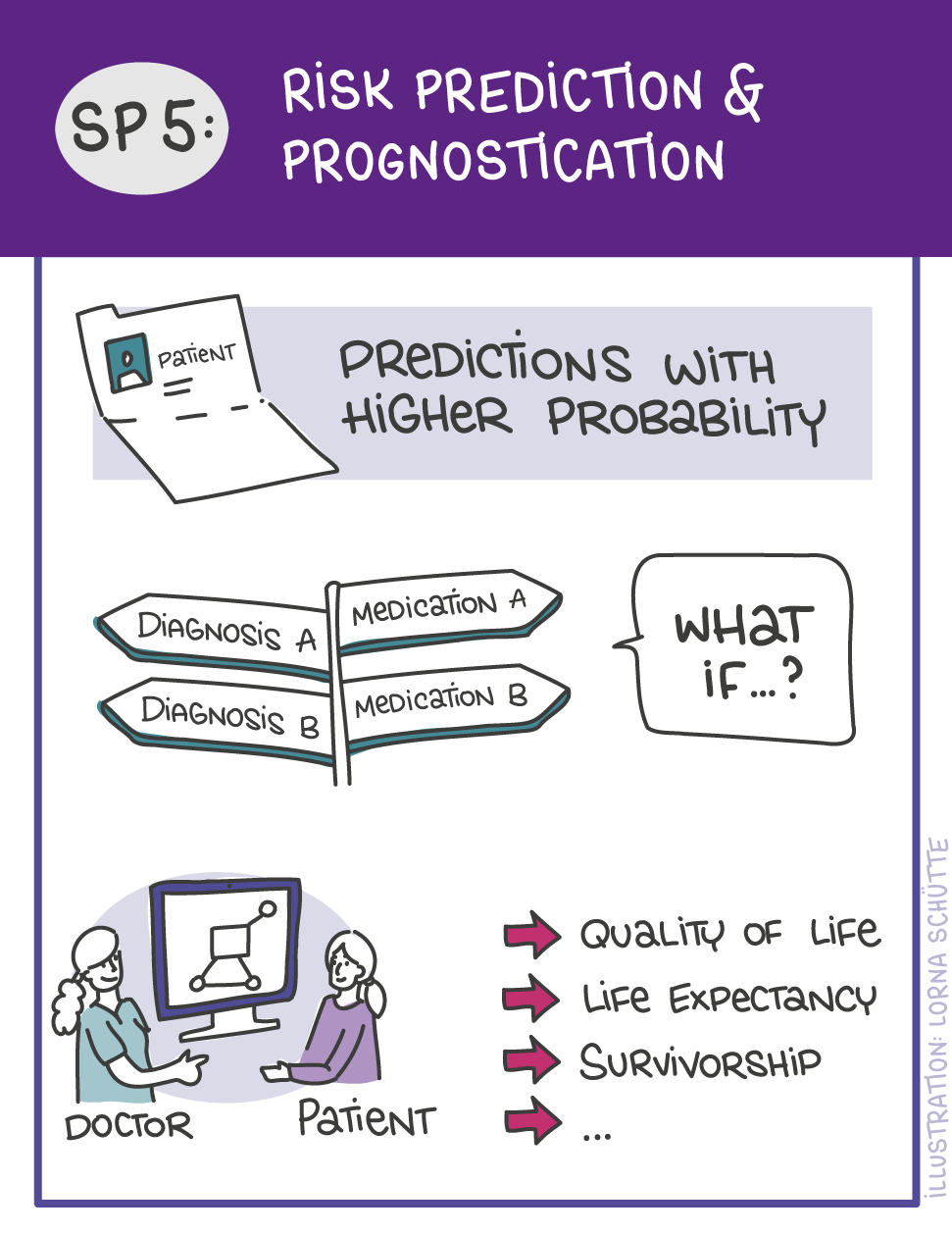
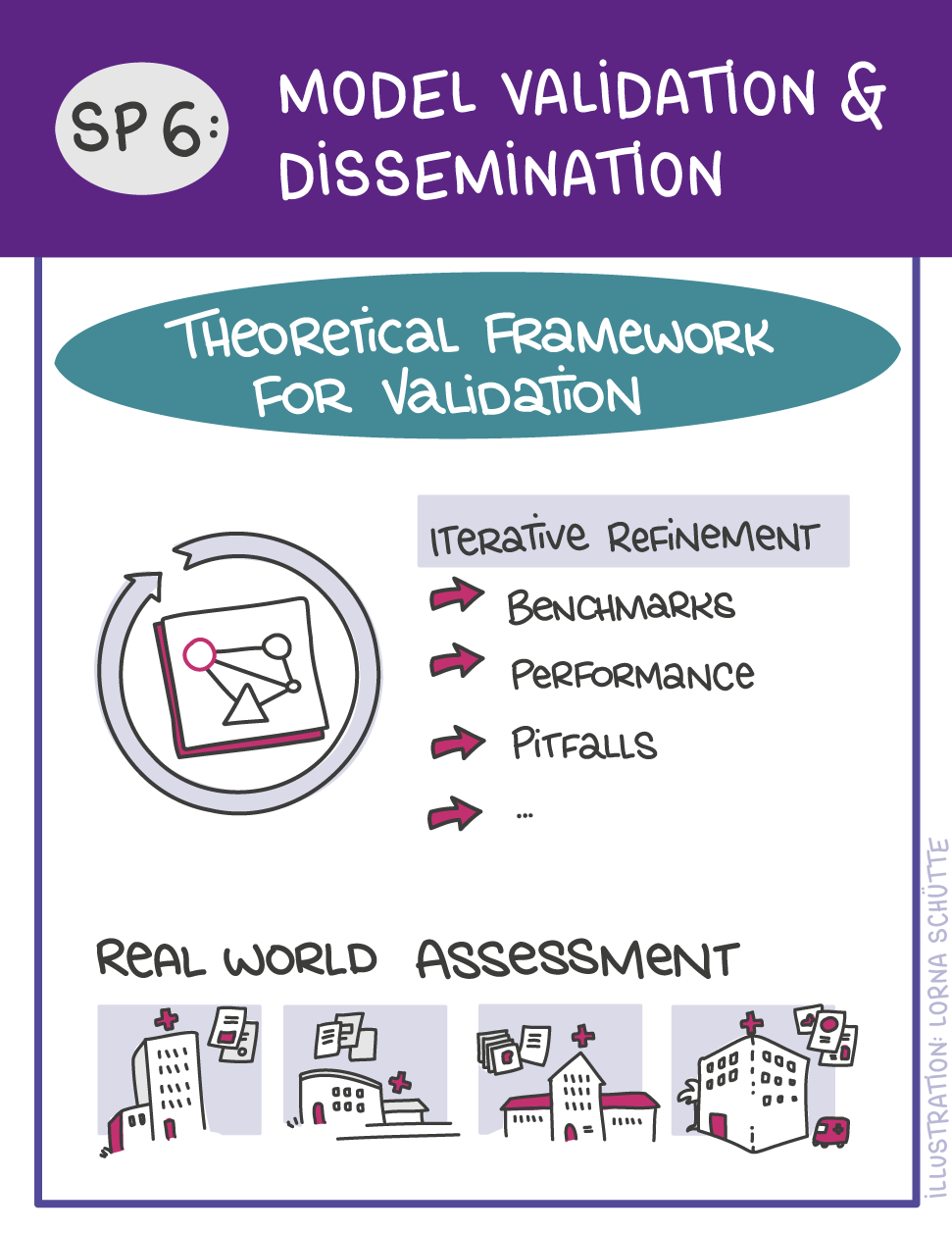
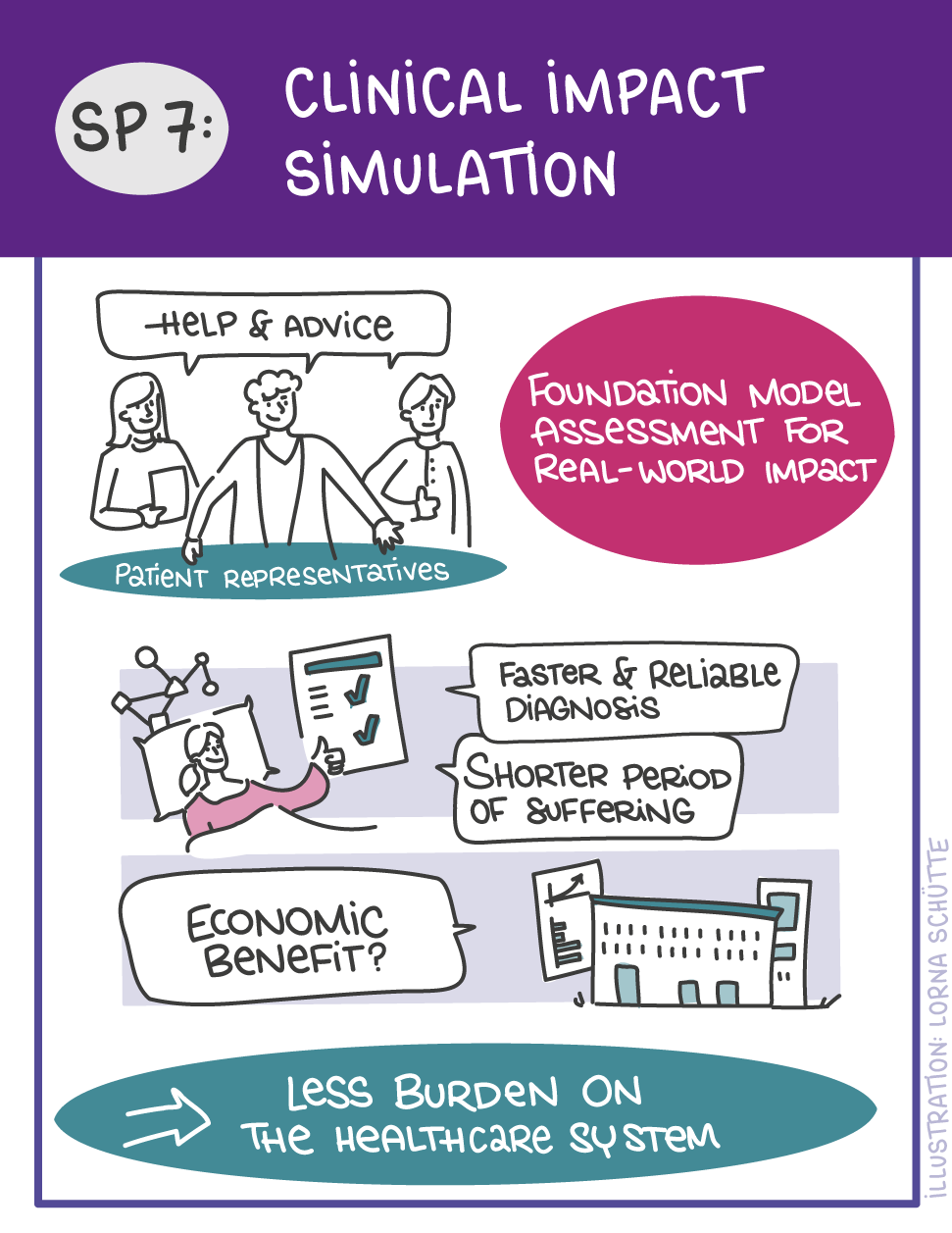
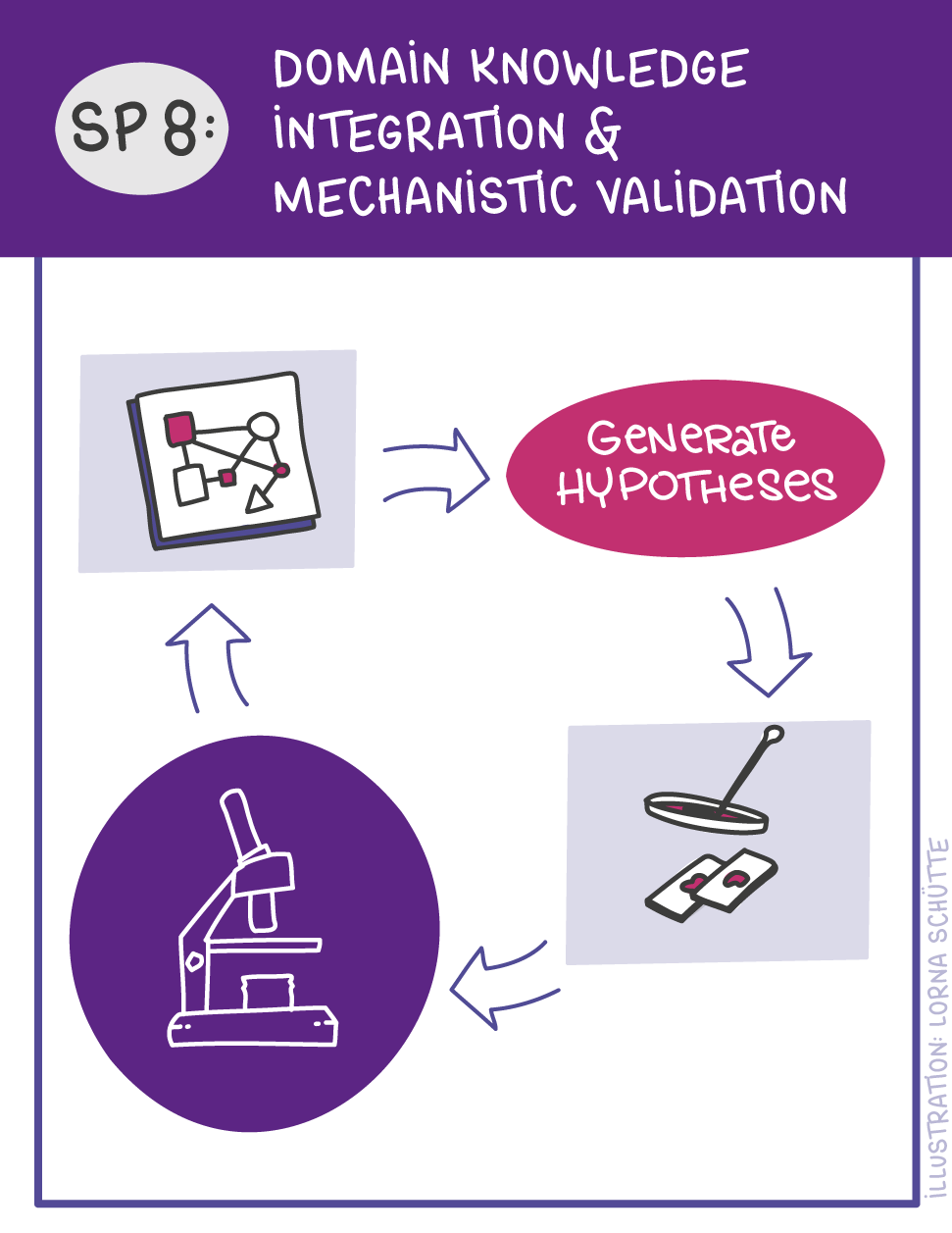
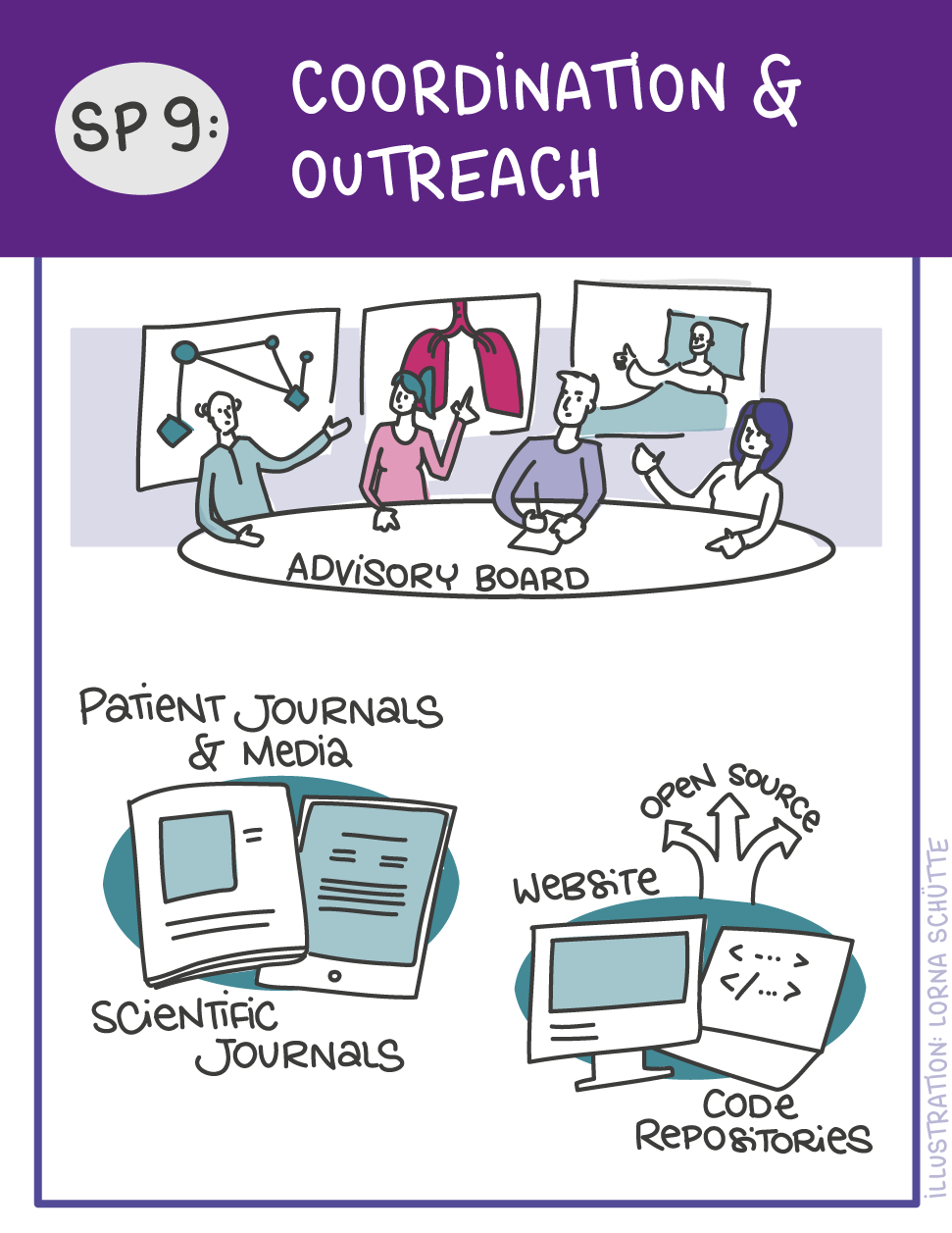
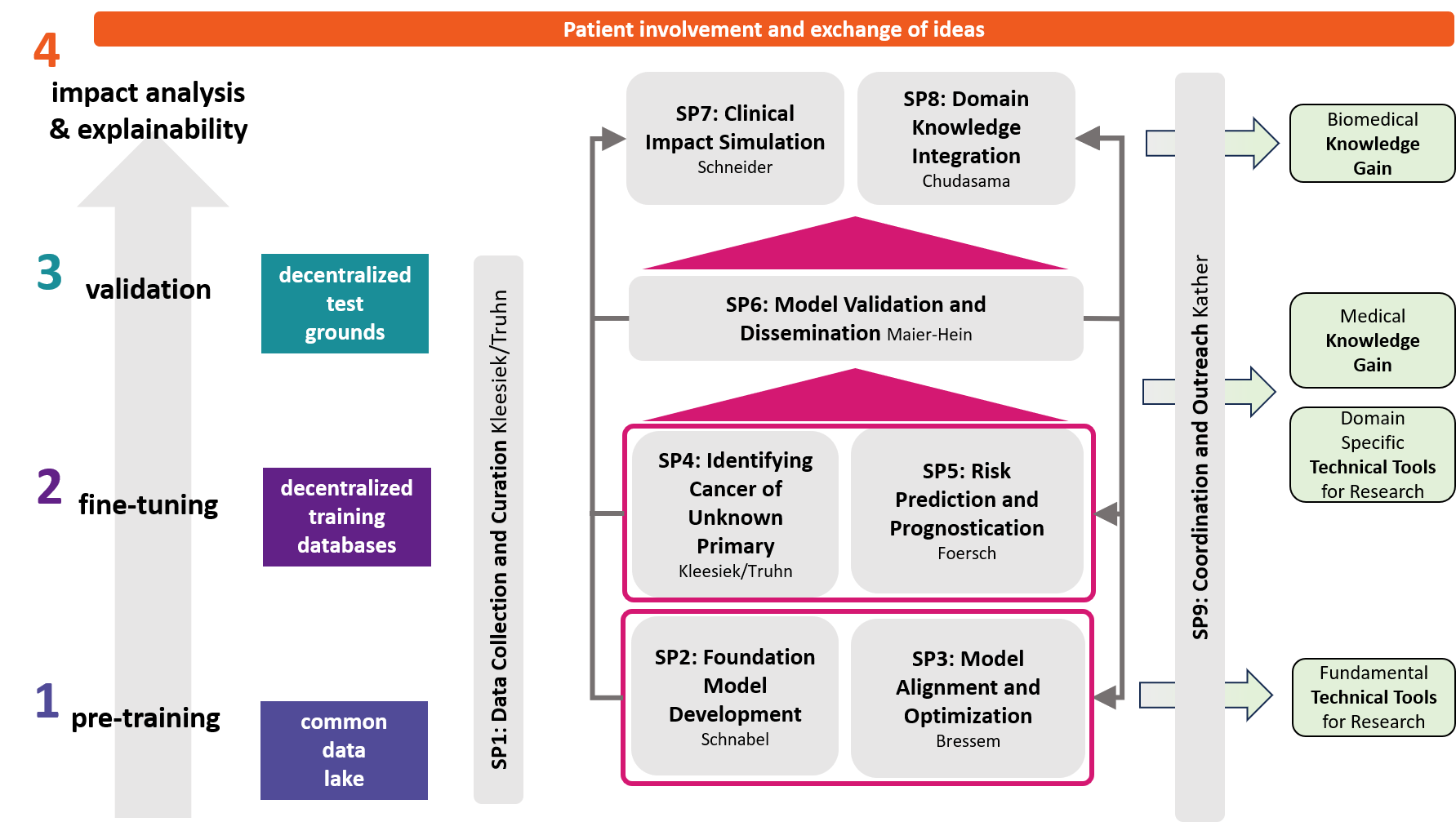
Patient Survey:
“Use of AI tools to better predict, detect and treat metastasized cancer”
Patient involvement is central pillar for our proposal. In order to incorporate patients’ expertise and perspectives into our research design from its very inception, together with the participating POs, we created a survey investigating different aspects of metastasis affecting the patients. These included, but were not limited to disease characteristics, clinical experience as a cancer patient, social and emotional aspects including quality of life while living with fear or experience of metastatic disease. Importantly, we also queried the current standpoint on use of artificial intelligence in clinical decision-making.
The survey was disseminated by all participating patient organizations (POs) in Germany and the Netherlands via mailing lists and social media, as well as through professional networks of participating investigators of the consortium. Within a short span of two weeks, a staggering amount of responses were received (n=650) from patients affected by diverse cancer types covering all age groups from adolescent and young adults to elderly patients. The willingness to participate and give a detailed description of personal experience wherever possible highlighted enthusiasm as well as unmet needs of the patients.
The results of the survey highlighted following key aspects:
- Strength of outreach capacities of our partner patient organizations.
- High metastasis burden in common and rare cancer entities, including survey responders experiencing metastatic lesions in hundreds and large lesions at the time of diagnosis.
- About 50% of responders were sarcoma patients, underlining unmet medical need in this large and heterogeneous group of rare cancers.
- Profoundly negative experience around clinical monitoring of the disease, mainly associated with uncertainty of diagnosis, long waiting times, treatment side effects, delayed detection of metastasis in spite of multiple visit to the clinics and absence of specialized care
- Significantly impaired quality of life due to fear of relapse and metastasis, especially before clinical imaging scans.
- A thoroughly positive viewpoint on implementation of AI-assisted clinical care and willingness to share own comprehensive clinical data for this purpose.
- Skepticism around AI-assisted clinical care in a minority of patients attributed to missing information, highlighting the need for timely education and dissemination of these approaches for effective clinical implementation.
Multiple aspects of the survey results were immediately actionable in the building of the proposal and have been implemented and mentioned accordingly. As elaborated in the proposal, we will involve patients as true partners of our research, and work with them to leverage the newest generation of AI-based foundation models and biological validation to push improved detection, risk prediction and tailored treatment strategies for cancer patients.
Detailed results of the survey are available for download here.