Swarm learning for improved
Researchers from the ODELIA project including Prof. Jakob N. Kather and his team at EKFZ for Digital Health, have recently published a key paper, addressing the growing challenge of analyzing increasing volumes of magnetic resonance imaging (MRI) data. The study, published in Nature Communications Medicine, explores how Artificial Intelligence (AI) can help manage the rising demand for breast cancer diagnostic imaging.
Oliver L. Saldanha, Jiefu Zhu et al.: Swarm learning with weak supervision enables automatic breast cancer detection in magnetic resonance imaging. Nature Communications Medicine 5, 38 (2025)
With new breast cancer screening guidelines set to recommend MRI for a broader group of patients over the next five years, the amount of imaging data to be analyzed will increase substantially. This presents a considerable challenge for radiologists who are already working under heavy workloads. However, AI offers a promising solution to decrease this load. The development of AI models, though, has been hindered by the need for manual annotations and stringent data-sharing regulations.
In response, the ODELIA project presents Swarm Learning (SL), a novel approach with an integrated pipeline that combines weakly supervised learning to reduce the need for detailed annotations with a technique that enables local model training while avoiding the need for centralized data sharing. The study used three datasets, with more than 1,300 breast MRI exams from institutions in the United States, Switzerland, and the United Kingdom, to train AI models. These models were then validated on two external datasets, with over 600 breast MRI exams from Germany and Greece.
The results of the study were promising. When SL was implemented in a real-world setup across three international centers, the models that were collaboratively trained outperformed those trained locally. Even with smaller datasets, the study demonstrated the feasibility of deploying SL internationally. An SL approach retains on-site data processing, addressing key challenges such as data privacy and variability in annotations.
The study from the ODELIA project highlights how collaboration between institutions can improve diagnosis, shown by the distributed datasets resulting in more effective AI training. This approach eliminates the need for detailed annotations and centralized data sharing, offering a solution that could help accelerate the development of AI in medical imaging. ODELIA’s research paves the way for future advances in medical AI, as demonstrated by the breast cancer screening use-case, while adhering to privacy standards and overcoming the challenges of large-scale data analysis.
More information about the project is available here ODELIA and at: odelia.ai
Read more about Prof. Kather’s research: Clinical AI
This news article was first published at: https://www.eibir.org/
This project has received funding from the European Union’s Horizon Europe research and innovation programme under grant agreement No 101057091.
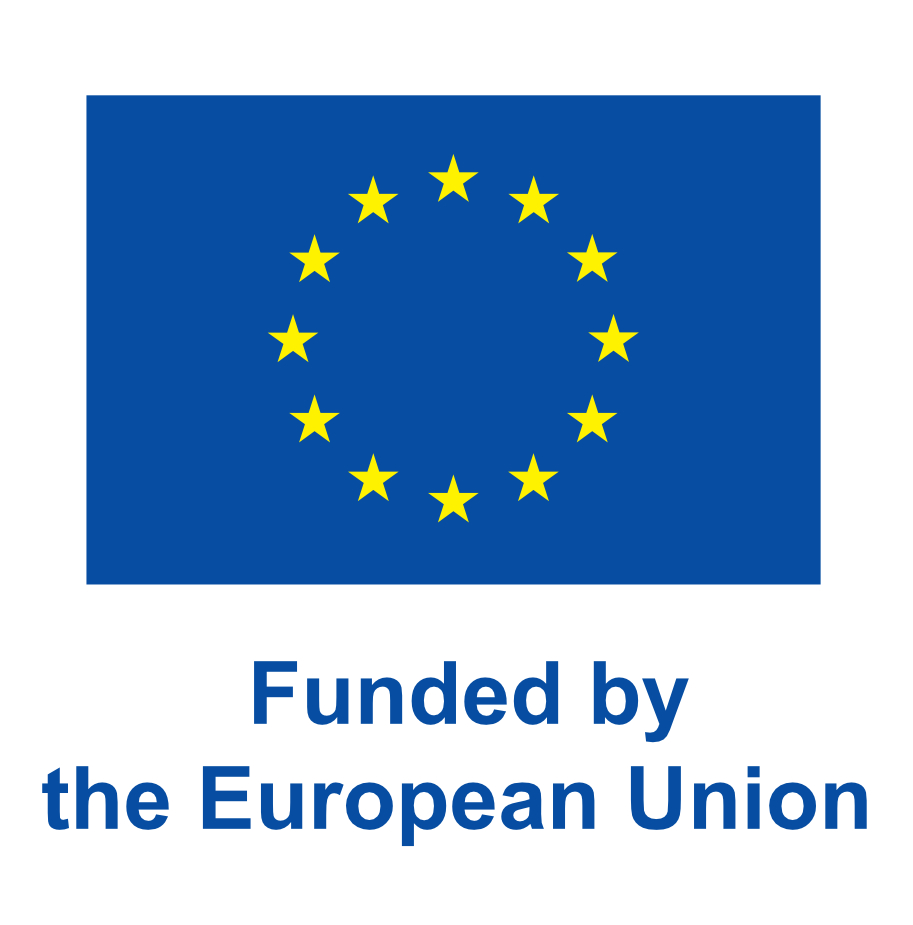
More News
Femtech, Ethics & Innovation: Highlights from the HALL-EKFZ Summer School 2025