Femtech, Ethics & Innovation: Highlights from the HALL-EKFZ Summer School 2025
Researchers show how Large Language Models can improve healthcare in the future
Much of the data that is collected on a daily basis in medical care is unstructured in the form of texts such as reports and doctor’s letters. In addition, extensive patient records are not fully digitized, depending on the hospital or practice. This is where large language models (LLMs) could help to reduce the workload of medical staff in the future. Researchers from Dresden led by Prof. Dr. med. Jakob N. Kather and Dr. med. Isabella C. Wiest, together with other scientists, have shown in two publications how this form of artificial intelligence (AI) could improve the quality of healthcare and research.
Facilitating the diagnosis of acute complications of liver cirrhosis
A serious complication of liver cirrhosis is acute decompensation, a sudden and severe failure of liver function caused by chronic damage of liver tissue. This needs to be quickly recognized and treated. The researchers were able to show that the local language model “Llama 2” successfully identifies important clinical features of the disease from patient records. To this end, they compared the results of their AI model with the diagnoses of three blinded experts. The AI performed very well in the tests and showed a high level of accuracy. In addition, the methods used protect patient privacy and sensitive medical data is processed securely and in compliance with data protection regulations on the clinic’s internal servers.
Simplifying the documentation of endoscopic examinations
Complications occasionally occur during endoscopic examinations. For optimal aftercare, incidents must be documented precisely and in a structured manner. However, there is often a lack of time in everyday clinical practice. Reports are written in the form of free text, which makes structured analysis difficult. The use of LLMs also offers a promising solution here. Information on adverse events can be automatically extracted from procedure reports without changing existing documentation practices. This facilitates quality assurance and reduces the manual effort involved in documentation.
Making daily work easier for doctors – improving medical care and research
In the two studies described, the researchers could show that locally used LLMs are capable of extracting clinical information from free text with a high degree of accuracy. At the same time, these models preserve privacy and protect sensitive patient data. The use of local solutions with low hardware requirements lowers the barriers to implementation in practice. “In the future, these AI methods could support doctors in their decision-making and facilitate the documentation of medical information. Complete documentation and improved traceability would not only directly improve the quality of healthcare, but also medical research in the long term,” says Dr. Isabella C. Wiest, lead author of the studies, doctor at the University Hospital Carl Gustav Carus Dresden and researcher at the EKFZ for Digital Health at TUD Dresden University of Technology.
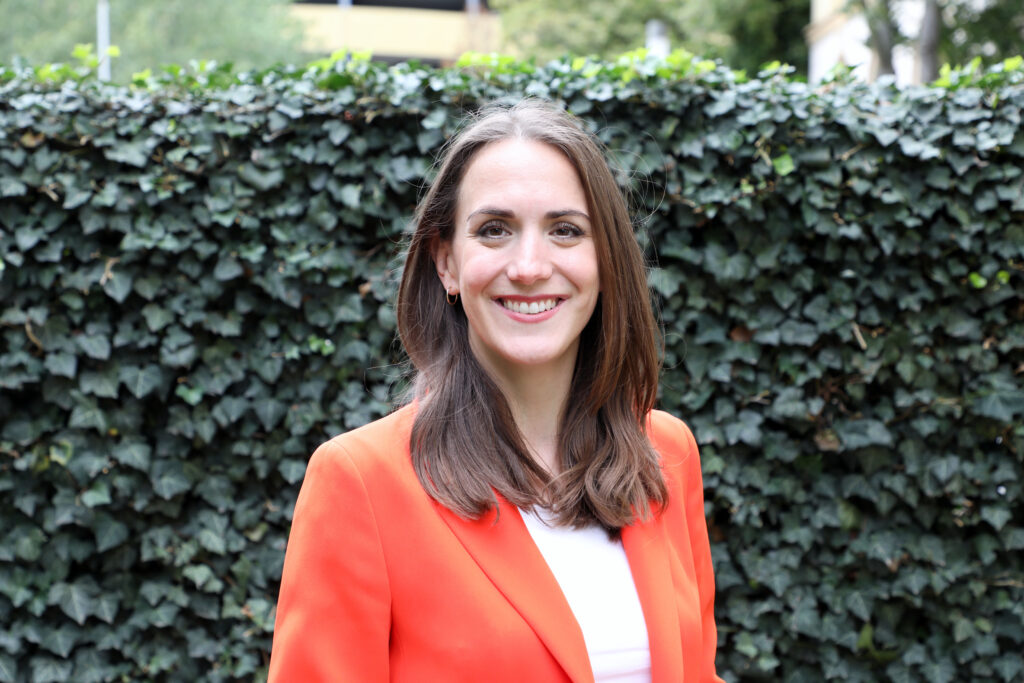
Isabella Wiest
In the future, these AI methods could support doctors in their decision-making and facilitate the documentation of medical information. Complete documentation and improved traceability would not only directly improve the quality of healthcare, but also medical research in the long term.
More News
Researchers describe potential weakness of popular AI models