How deep learning identifies key mutations in colorectal cancer for better treatment options
Nowadays, cancer medicine offers a variety of therapies depending on the specific tumor subtype. However, finding the right therapy for individual patients and predicting disease outcome can be challenging. Using artificial intelligence, the group of Professor Jakob N. Kather together with colleagues from Créteil/France, Augsburg, Heidelberg and Leeds/UK have published a method in the Nature portfolio journal “npj Precision Oncology”, which is able to predict specific genetic subtypes of colorectal cancer based on their appearance on histopathology slides.
Marco Gustav, Nic Gabriel Reitsam, Zunamys I. Carrero, Chiara M. L. Loeffler, Marko van Treeck, Tanwei Yuan, Nicholas P. West, Philip Quirke, Titus J. Brinker, Hermann Brenner, Loëtitia Favre, Bruno Märkl, Albrecht Stenzinger, Alexander Brobeil, Michael Hoffmeister, Julien Calderaro, Anaïs Pujals, Jakob Nikolas Kather: Deep learning for dual detection of microsatellite instability and POLE mutations in colorectal cancer histopathology; npj Precision Oncology, 2024.
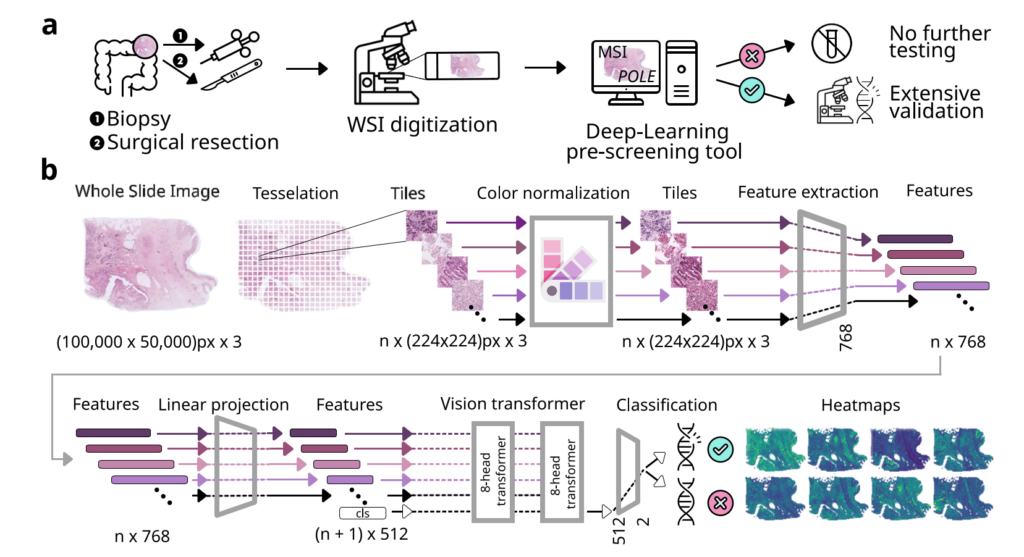
Experimental design and study overview.
Image taken from Figure 1 a-b, Gustav et al. 2024, npj Precision Oncology without changes under CC BY 4.0 Licence. https://doi.org/10.1038/s41698-024-00592-z
Colorectal cancer subtypes can be classified based on microsatellite stability (MSS) or instability (MSI). Microsatellites are short, repetitive DNA sequences distributed throughout the genome. In cancer, MSI occurs when these sequences become unstable due to defects in the DNA repair system. MSI serves as a key biomarker to identify patients who are candidates for immunotherapy, as only a small fraction of colorectal cancers responds to this type of treatment.
It has already been demonstrated that deep learning can directly predict MSI status from routine histology slides, providing a fast and cost-effective pre-screening tool for further genetic sequencing. Interestingly, a small subset of colorectal cancers shows MSS but may still respond to immunotherapy. These patients, with mutations in the DNA repair gene POLE, can potentially benefit from immunotherapy. However, because these mutations affect fewer than 1% of cases and require expensive genetic testing, they are rarely diagnosed routinely. Marco Gustav, a member of the Kather Lab, and other scientists have enhanced this pre-screening method. They trained a deep learning classifier on a large dataset and validated it on three external cohorts to accurately identify the MSI status of colorectal tumors from standard histological data. Notably, cases flagged as MSI by the model but shown to be MSS in routine sequencing might harbor POLE mutations, which can then be prioritized for sequencing.
In a clinical setting, this model could serve as an efficient preliminary screening tool to identify clinically relevant MSI and POLE mutations in colorectal tumors.
The publication in npj Precision Oncology was created in collaboration between researchers from the EKFZ for Digital Health, Dresden University Hospital Carl Gustav Carus, University of Augsburg, DKFZ and NCT Heidelberg, University Hospital Heidelberg, Leeds Institute of Medical Research at St James’s, University of Leeds/UK, Université Paris Est Créteil, INSERM, IMRB, Assistance Publique-Hôpitaux de Paris, Créteil/France.
More News
VisionMed: Fellows of the Roland Berger Foundation experience smart solutions for medicine